Implementing self-service data – practical lessons from Opendatasoft
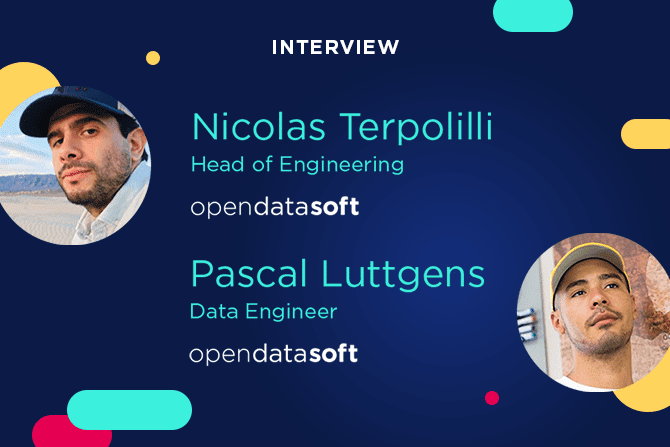
How can you successfully introduce self-service data sharing across your organization? In this blog we share best practice from Opendatasoft’s own self-service project, providing tips and advice based on our experience to date.
Many organizations have plans to share information internally and externally, democratizing data access. But how can they turn their vision and strategy into action and what challenges do they need to overcome?
At Opendatasoft (ODS) we’re committed to data democratization across the organization, and have embarked on our own self-service data project. To find out more and the lessons we’ve learnt, we spoke to Nicolas Terpolilli, Head of Engineering, and Pascal Luttgens, Data Governance Engineer, to get their feedback from implementing self service data for our teams.
Internal self-service data sharing at Opendatasoft
Our internal data sharing portal brings together data from all departments, allowing us to track activity across our platform and make it available to the entire company.
What were the objectives behind ODS’s self-service data project?
Pascal: As an organization Opendatasoft generates and uses a lot of data. However, while we have a data culture, and everyone understands the value of data, information was mainly shared and governed on a team level. As we grew larger we saw the opportunity to put in place data governance on a company-wide level, providing self-service data to those that needed it.
And of course, with our platform we have the perfect solution to our problem. The experience we gain implementing self-service data internally will not only help us, but will also be useful for our customers as they undertake similar self-service data projects.
Were there any particular pain points you were looking to solve?
Nicolas: The main pain point was that, as with most organizations, different departments use different data and tools. This is perfectly natural as each one has been chosen to be the best fit for their particular needs. For example, we use Salesforce for business and CRM data, and custom software we developed internally to administer our platform.
This meant that customer data was spread between the two solutions – which made it difficult to provide a complete view of the customer to everyone involved, such as Customer Success Managers, and technical support,.
We therefore wanted to build a bridge between Salesforce and our administration platform software to enable transverse data sharing on a self-service basis. This was especially to help the support team as they need accurate, up-to-date business information when working on the ground. If they get an email or support ticket from a customer then they need to know everything about them so that they can make more informed decisions when talking to them. So the overall aim is about data democratization – trusting people to make better decisions by giving them the information they need to be independent and effective.
All of this helps deliver real benefits – a better experience for customers, faster operations internally, and greater productivity, together contributing to higher revenues.
What architecture are you using?
Nicolas: We want to remain flexible and agile, with a decentralized approach to data. That led us to choose a data mesh architecture. As my colleague David Thoumas explains in this blog, data mesh makes it faster and simpler to share data and to achieve true data democratization.
It offers many advantages for us – for example, in data mesh the departmental owners of data (such as the support team) are data stewards responsible for quality, backed up by company-wide governance and standards. And as data mesh doesn’t rely on specific tools or technology, we have the flexibility for everyone to use the tools they are most comfortable with, helping increase adoption.
Where are you now and what are the next steps on the project?
Pascal: We now have an internal data platform that has a mix of operational data, analytical data and information people need about our solution from our administration platform. There are 90 users currently and over 20% of the company has published a dataset of some sort. Overall this means we have between 50-100 datasets, though over time we want to increase this by adding more operational data. We’re now designing the infrastructure to share all of this data effectively.
While some teams, such as customer success, are already on board and actively using the platform for data sharing others need more support and training. So our next step is to acculturate everyone to ensure that they are aware of what data mesh is and what it means to their working lives. It’s much more about culture than technology.
We’re going to present this concept to the company, along with governance guidelines. These will show who is in charge of which data, the resources that are available and our overall processes around data. The aim is to break down silos internally and increase data sharing. On the technical side we’re also going to improve the analytics side of the self-service platform, adding connections through our APIs to business intelligence tools.
What best practice advice do you have for other organizations?
Nicolas: We’ve really learned a lot so far during the project, less about technology but more about culture and governance. I’d highlight these six best practice tips:
- Start by identifying who is in charge of different datasets.
- Gain agreement on a common language to describe data. For example the word “customer” means different things to different teams within ODS. It means a project within a customer to a customer success manager, but an account to business people.
- Start small and go fast with limited data – we started with the customer success and sales teams. Take a step by step approach. For example, publish a basic page and a dashboard early to build trust. You can correct any errors and get buy-in when it is still small. Don’t build everything and then do a big unveil as you risk rejection.
- Recognize that bringing data together will break down silos and drive greater transparency. That’s a big cultural shift – for example, everyone can see how other departments are progressing against their objectives/targets.
- Understand that the project will highlight processes that can be improved as you can now compare different data. Being able to fix these problems is a clear benefit of the program, so uncovering them should be seen as a positive. In our case we can now discover issues extremely fast due to the project.
Pascal: I’d add two more points:
- When it comes to using technology people focus on the experience, ease of use and the design of dashboards, rather than the data itself. So make sure you factor that in – our platform is very simple to customize so we can tailor it to user needs. Remember you are changing people’s daily workflows so if you want them to use the platform remove obstacles such as poor UX. If they are used to a good experience don’t take a step backwards.
- If people have existing reports/dashboards that they rely on, then copy these across so they can do things in the same way first. Then expand to give them more powerful options – success is all about taking the users with you.
As our project progresses we’ll keep providing updates on key milestones. In the meantime, find out more about how our platform enables self-service data sharing by clicking here.
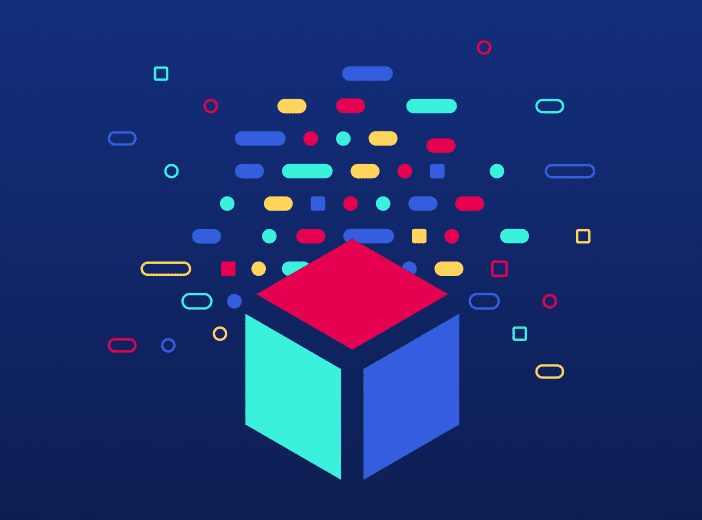
It can be hard to understand exactly what a data product is, given the many ways that the term is defined and applied. To provide clarity this article provides a business-focused definition of a data product, centered on how it makes data accessible and usable by the wider organization, while creating long-term business value.