Glossary
Data science
Data science is the practice of extracting and applying valuable information and insights from large volumes of structured and unstructured data.
What is data science?
Data science is the practice of extracting and applying valuable information and actionable insights from large volumes of structured and unstructured data. It uses a combination of advanced analytics techniques, artificial intelligence algorithms, software, and scientific principles to achieve this aim. The knowledge extracted during the data science process underpins data-driven decision making, strategic planning, and predictive analysis.
Data science is often used as an umbrella term to describe all activities related to collecting, managing, analyzing, understanding and using data. However, data science teams do not always oversee all parts of the data lifecycle – for example IT may be responsible for collecting and preparing data on a technical level, while business analysts query data and produce reports and dashboards to deliver insights to organizations.
How does data science differ from business intelligence?
Both business intelligence (BI) and data science aim to improve decision-making through data analysis. However, BI focuses on descriptive analysis of structured, historic data. It can explain what is happening in the company and market, such as providing quarterly sales figures for specific products.
By contrast data science uses more advanced analytics, analyzing a wider range of structured and unstructured data sources. It enables the use of predictive analytics that forecast future behavior and events, delivering foresight to prepare for potential scenarios.
Why is data science important?
Understanding and harnessing data is crucial to competitiveness in all industries, particularly as the amount of available data has grown exponentially. Data science is therefore a vital activity for organizations in order to:
- Underpin more informed decision-making, based on data rather than guesswork
- Better understand customers and deliver products and services to meet their needs
- Optimize operational efficiency by improving processes
- Reduce risk, detect fraud, and ensure regulatory compliance
- Improve supply chain management through accurate forecasting
- Predict future trends, particularly through AI, enabling businesses to out-perform rivals
- In healthcare, improve diagnoses and provide early warning of potential illnesses
What is the data science process?
Data science normally follows a five-stage life cycle:
- Capture/ingestion —gathering raw structured and unstructured data from multiple sources.
- Maintain/store — storing, cleansing, and processing data to make it usable.
- Process — mining, classifying, modeling and summarizing data.
- Analyze — analyzing data to test hypotheses and extract relevant insights.
- Communicate — sharing and reporting the results with business users through understandable reports, data visualizations, dashboards and charts.
What does the job of a data scientist involve?
Data scientists specialize in extracting and applying actionable insights from data. They are normally skilled in detecting patterns hidden within large volumes of data. Normally operating in teams, successful data scientists require a mix of skills and attributes:
Data scientists need knowledge and skills in computer science, statistics, information science and database management, math and modeling, creating compelling data visualizations, AI/machine learning algorithms and programming languages such as R, Python and SQL.
They also have to be:
- Understanding – business understanding of their organization and its aims
- Curious – always thinking “what if?”, combined with an eagerness to ask questions
- Critical thinking – the ability to make informed decisions based on analytical results
- Collaborative – the ability to work closely with others within the data science team
- Communicating – the ability to share their findings in compelling ways with non-specialist audiences
What are the challenges to implementing a data science strategy?
Data science is a relatively young discipline and is new to many organizations. Programs face four challenges to success:
- Volume and complexity of data: Organizing and standardizing the sheer amount of data, from multiple sources and in different formats can be difficult, leading to an incomplete picture of the data landscape.
- Finding the right skills: Data scientists are in heavy demand, with only a finite number of people having the right combination of skills, experience and attributes. Recruitment can therefore be a challenge, particularly within organizations outside the technology sector.
- Access to the right tools: Data science requires an integrated technology stack that addresses all stages in the data science process, from ingestion to communication. This can be expensive to create, while ensuring that tools work together and meet organizational needs also requires planning, training and time.
- Disconnect with the business: The role of data science is to support the business and to help it remain competitive. However, it can become a siloed, research function that is disconnected from the business and its needs and is not seen to deliver quantified business value..
Learn more
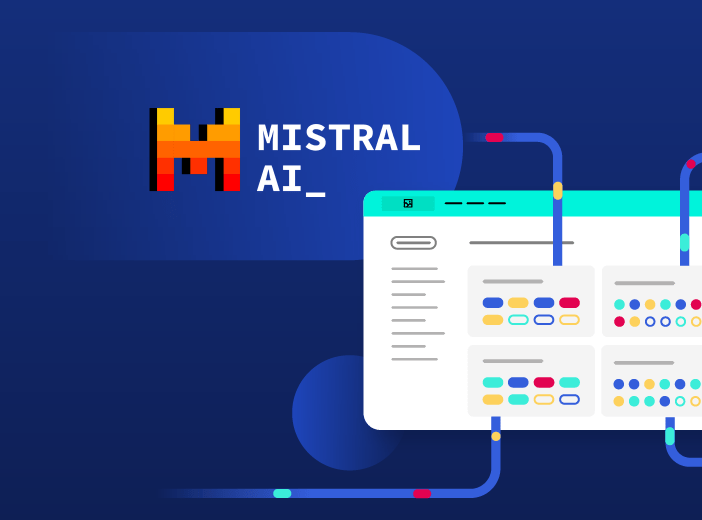
Opendatasoft integrates Mistral AI’s LLM models to provide a multi-model AI approach tailored to client needs
To give customers choice when it comes to AI, the Opendatasoft data portal solution now includes Mistral AI's generative AI, alongside its existing deployment of OpenAI's model. As we explain in this blog, this multi-model approach delivers significant advantages for clients, their users, our R&D teams and future innovation.