The 4th and Last White House Open Data Roundtable: Open Data for Public-Private Collaboration
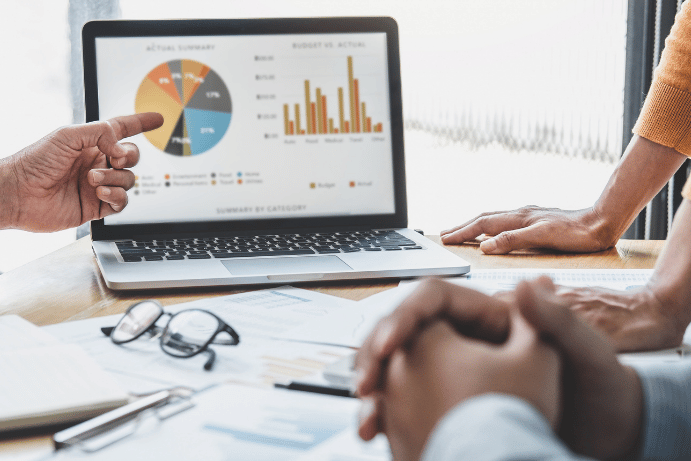
As we saw in our recap of the 2nd White House Open Data Round Table, data quality is crucial to Open Data. But looking further than just this one massive subject, it is also crucial to the growth of Public-Private collaboration through data interoperability.
The White House releases Open Data in the context of an asset. “Data as an asset” is encoded on the White House policy of “open by default” detailed in memorandum M13-13. To make possible the release of higher value Open Data to the public, the White House Office of Science and Technology (OST) in conjunction with the Center for Open Data Enterprise, convened four Roundtables. This last Roundtable was on Public-Private Collaboration.
Open Data as an Asset
The Center for Open Data Enterprise’s own page on the Roundtables states their objectives:
- Identify Open Data case studies, learned lessons, and best practices across the Federal Government;
- Strengthen a community of technical, legal, and policy experts in support of Open Data;
- Support continuity and accelerate the progress of Open Data work.
The Center convened a community of experts from the Public and Private sectors. As one of them, I was able to learn about the state of Open Data at the Federal level and see first hand these examples of success. All documentation and discussion that I have seen boil down to data quality and data discoverability. We are not there yet. Open Data is hard to find and difficult to use. The quality is often a barrier.
My conclusions after the Fourth White House Open Data Roundtable:
- The Center for Open Data Enterprise and the White House OST both agree there are areas of opportunity for improving data governance in the US;
- Both organizations have consulted with private sector companies, including Opendatasoft, to understand best practices around data governance;
- Everyone agrees that the quality and provenance of the data is at least as important as the data.
Data Quality and Data Provenance are Interconnected
Let’s unpack this last statement. How can the quality and the provenance be as important that the data? Quality is just what it sounds like. How does one determine whether data are quality or not? In my previous blog post we alluded to some of the characteristics of data quality. One of the participants of the second Roundtable asserted that quality should be proportional to use. How much of an impact are these data capable of? That is the extent to which quality should then be applied.
Definitions of Data and Data Quality
The Second Roundtable (April 27th, 2016) had the following definition of data quality. Data are measured by:
- Completeness;
- Validity;
- Accuracy;
- Consistency;
- Availability;
- Timeliness.
There is also the “official” explanation of data quality. (See ISO 8000 the International Standard for Data Quality).
First ISO 8000 defines “Data”
“Data: reinterpretable (reusable) representation of information in a formalized manner suitable for communication, interpretation, or processing”. (See ISO/IEC 2382-1:2015)
Then ISO 8000 defines “Data Quality”
Data quality is defined as having: Syntax; Semantics (metadata); Source of data (provenance); Fitness; Accuracy; Completeness. (See ISO 8000: Master Data Quality part 120).
This is not the only source for definitions on data quality but this is one of the more complete definitions. The W3C has announced a draft of Open Data Standards which includes a section on normalization and standardization regarding metadata and data discoverability. (See Data on the Web Best Practices. W3C Working Draft 19 May 2016).
Private sector actors like OpenDataSoft, ESRI and Socrata all agreed that the quality and provenance of the data is at least as important as the data. This was shown on a whiteboard at the end of the Roundtable.
Other Discussions on Data Quality
- Martin, Erika G., et al. “Evaluating the Quality and Usability of Open Data for Public Health Research: A Systematic Review of Data Offerings on 3 Open Data Platforms.” Journal of Public Health Management and Practice (2016).
- Batini, Carlo, and Monica Scannapieco. “Data Quality Issues in Linked Open Data.” Data and Information Quality. Springer International Publishing, 2016. 87-112.
- Ruan, Tong, et al. “Kbmetrics-a multi-purpose tool for measuring quality of linked open data sets.” The 14th International Semantic Web Conference, Poster and Demo Session. 2015.
- Kubler, Sylvain, et al. “Open Data Portal Quality Comparison using AHP.“Proceedings of the 17th International Digital Government Research Conference on Digital Government Research. ACM, 2016.
- Pulla, Venkata Sai Venkatesh, Cihan Varol, and Murat Al. “Open Source Data Quality Tools: Revisited.” Information Technology: New Generations. Springer International Publishing, 2016. 893-902.
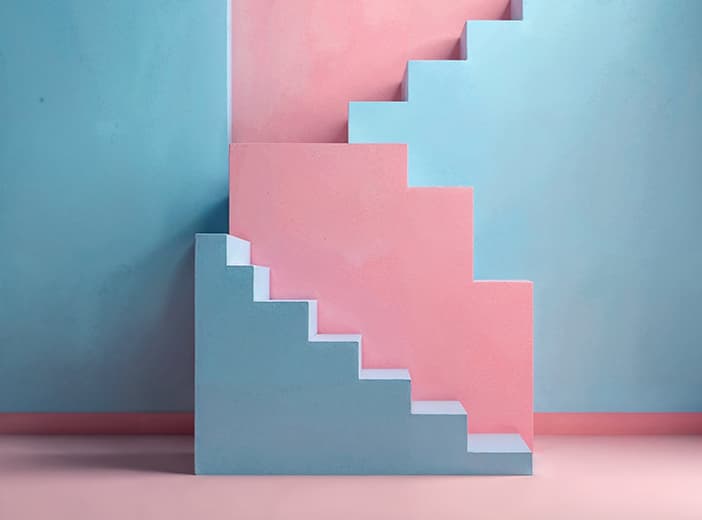
How successful are governments at sharing their data with citizens and businesses? The latest Open Data Maturity report provides an overview of progress across Europe, and highlights the importance of improving data portals and measuring impact to future success
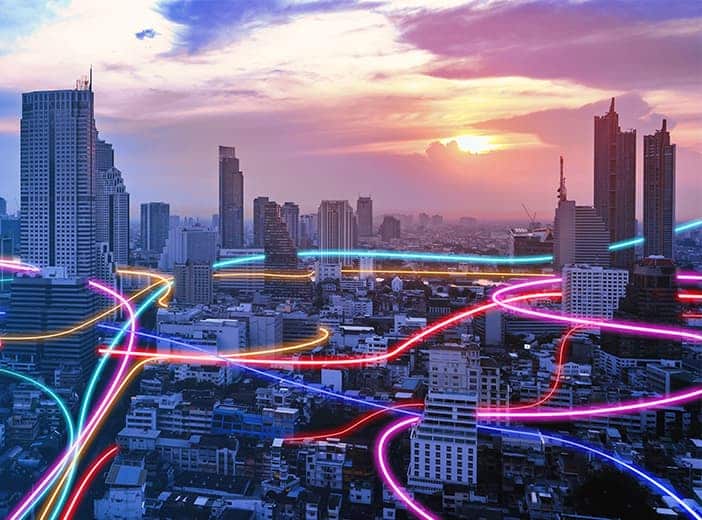
Data drives effective, well-functioning smart cities and helps build local ecosystems that bring together all stakeholders to meet the needs of the entire community. However, sharing data between stakeholders can be difficult - based on recent Gartner research we explain how urban data exchanges transform smart city data sharing.