Glossary
Extract, Transform, Load (ETL)
Extract, Transform, Load (ETL) is the data integration process used to combine data from multiple sources into a single, centralized repository.
What is Extract, Transform, Load?
Extract, Transform, Load (ETL) is the three stage data integration process used to combine structured and unstructured data from multiple sources into a single, centralized repository, such as a data warehouse, data mart, or data lake. The three steps involved are:
- Extract: Raw data is accessed and moved from its source system to a staging area
- Transform: In the staging area business rules are applied to cleanse, transform and organize the data to ensure quality and consistency
- Load: The data is then added to the repository
Carried out using software tools, the ETL process can be automated to take place on a regular basis, through batch updates or in real-time when data in a source system changes. These ETL pipelines (or data pipelines), bring together tools and activities to automate data integration, increasing efficiency and enabling them to be replicated, monitored and improved.
ETL vs ELT
ETL has been in use since the 1970s. More recently it has been joined by Extract, Load, and Transform (ELT). As the name suggests, this reverses the order of the second two steps, loading data into the target repository, where it is then transformed. This removes the need for a staging area, and adds flexibility to data analytics. This means it works well for high volume, unstructured big data, such as that stored in data lakes.
However, it requires central repositories both to have data mapping capabilities and access to sufficient processing power to successfully carry out the transformation step efficiently and quickly. This makes it best deployed in the cloud, which provides access to the scalable, on-demand computing power required to undertake transformations.
Why is ETL important?
Structured and unstructured data is now produced by multiple systems inside and outside the organization, in a large number of different formats. To create value this data needs to be made available widely, especially to underpin business intelligence and enable better, data-driven decision making. This requires it to be centralized and standardized to ensure accessibility, quality and governance. Extract, Transform, Load processes enable this, making them central to:
- Effective, informed data analysis and reporting through a consolidated view of information
- Ensuring data quality and governance by applying consistent standards
- Delivering a single version of the truth for everyone
- Providing historical context for business intelligence
- Increasing efficiency by replacing manual processes
What are the steps in Extract, Transform, Load (ETL)?
Extract
In the data extraction phase, raw data is copied or exported from source locations to a staging area. Data can be structured or unstructured and can come from sources such as relational databases, data storage platforms, cloud storage, or business applications (such as CRM or ERP systems).
Transform
This is the most important part of the ETL process, as it ensures that the raw, extracted data is checked and transformed in the staging area so that it meets corporate guidelines around standards, quality and accessibility.
Data transformation normally includes:
- Cleansing — removing/resolving inconsistencies and missing values
- Standardization — applying standard governance rules to the dataset
- Deduplication — discarding redundant or duplicate data
- Verification — removing unusable data and flagging anomalies
- Encrypting/protecting data – such as by anonymizing sensitive data
- Sorting — organizing data by type
- Improving – enriching datasets with additional data (such as reference data) to increase its value
Load
Once the data is transformed, it is then loaded into a centralized repository, such as a data lake or data warehouse. All data can be loaded at once (full load) or at scheduled intervals/when a record changes (incremental load). Typically, this final stage takes place outside peak business hours when traffic on the source systems and the data warehouse is at its lowest.
Learn more
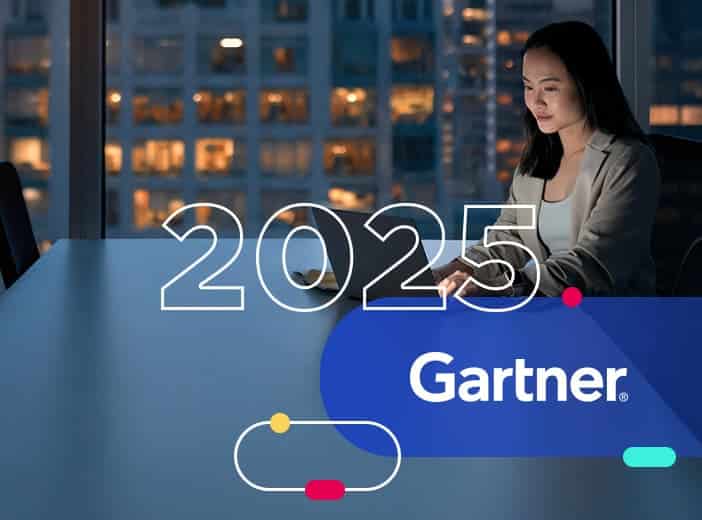
How data leaders deliver value – Gartner’s 2025 Leadership Vision
The role of the data leader has never been more vital, but they face growing pressure to increase their business value and support AI initiatives. Based on a new Gartner guide, we outline the vision to follow to maximize success for their organization.