Glossary
Data Lifecycle
Data plays an essential role in organizational success. It fuels strategic and operational decisions. It drives innovation and revenue generation for those who implement effective data management processes to fully exploit its value. To achieve this, it is essential to master all stages of the life cycle. Data is not static: it follows a dynamic path from creation to sharing - the key stages that constitute the data lifecycle.
The Stages of the Data Lifecycle
Optimal data management takes into account each of the following stages:
1.Data Collection
Data collection is the first stage of the data lifecycle. Data can be collected through various methods:
- Use of pre-existing data within the organization
- Manual entry
- Online forms
- Web scraping
- Purchasing databases
- Automated collection through IoT sensors.
Collection relies on interfaces that require an exact match between the objects described in each field, their names, and the values associated with these fields.
The nature of this data may vary depending on its source: it may be related to the organization’s business system, be unstructured data (such as video files, audio, or word processing documents), partner data, or real-time data (such as from IoT sensors).
2. Data Storage
Data storage is crucial to ensure the security and future accessibility of data. It is important to establish a robust backup process to ensure data preservation throughout its lifecycle. Different types of storage solution can be used, such as relational databases, data lakes, data warehouses, and Cloud solutions to enable organized and reliable data storage, facilitating its subsequent use.
3. Data Processing
After storage comes the data processing stage. The first phase of this processing stage is data cleaning (sometimes called data cleansing). This involves cleaning, validating, transforming and structuring raw data to make it usable for analysis or modeling. The data is then compressed: its size is reduced, optimizing storage and transfer speed. Finally, data encryption ensures data security by making it unreadable to unauthorized individuals, thus guaranteeing sensitive information remains confidential.
4. Data Analysis
Data analysis involves extracting information and trends from raw data. Various tools are available to do this, including business intelligence, artificial intelligence, machine learning, and statistical modeling.
5. Data Sharing
Once analyzed, data must be shared with the organization’s internal or external stakeholders. This is best achieved via online data portals. These portals offer centralized access to data assets from various sources, thus facilitating data democratization.
6. Data Archiving
Data archiving begins with identifying inactive or unused data. This data is extracted and stored in long-term storage systems. This enables historical data to be preserved in order to meet regulatory compliance requirements or to allow organizations to conduct retrospective analysis.
7. Data Deletion
The data deletion process is implemented when the organization wishes to dispose of inactive or obsolete data. This approach has two advantages: it reduces both storage costs and the risks of non-compliance with prevailing regulations. Note that in the case of sensitive data, physical backup media must also be securely destroyed.
Best Practices to Apply throughout the Data Lifecycle
To fully leverage its data assets, each organization must consider best practices to apply to each stage of the data lifecycle:
Data Governance
Data governance is essential to ensure consistent, reliable, secure, appropriately used, and compliant data.
Security
Organizations must implement a set of measures and strategies to protect their data against potential threats such as cyberattacks, data breaches, and unauthorized access. Practical measures include data encryption, access management, monitoring suspicious activities, and user awareness training.
Regulatory Compliance
To ensure compliance when sharing data, it is imperative to establish uniform guidelines and procedures within a data governance program. These guidelines ensure that every individual handling data adheres to established internal and external standards. This includes regulations such as GDPR in Europe or CCPA and HIPAA in the United States. Organizations must implement specific policies and procedures to ensure that their data management practices comply with these regulations, to avoid legal sanctions, fines, and reputational damage.
Learn more
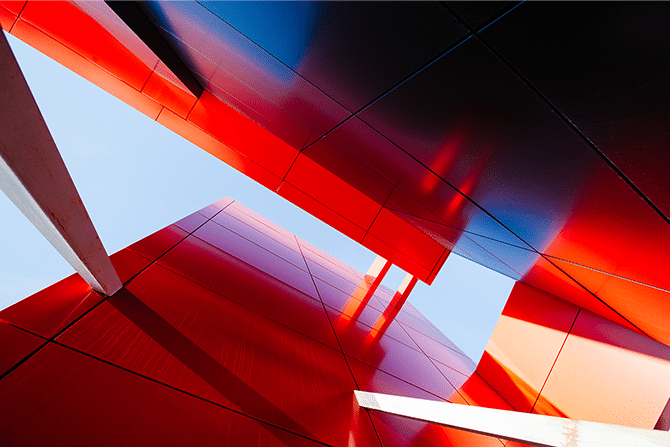
Data portals: The essential step to building an external data ecosystem
Extending data sharing across entire ecosystems drives transformative benefits in terms of efficiency, collaboration and innovation. While a new concept to many organizations, pioneers are already seeing the advantages of building external data ecosystems - we explore the foundations required, starting with intuitive, centralized data portals.
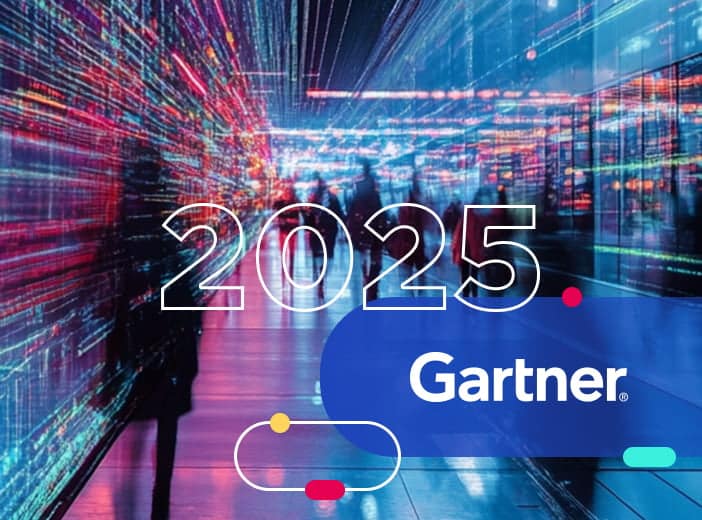
2025 data leader trends and the importance of self-service data – insights from Gartner
Growing data volumes, increasing complexity and pressure on budgets - just some of the trends that CDOs need to understand and act on. Based on Gartner research, we analyze CDO challenges and trends and explain how they can deliver greater business value from their initiatives.