7 key ways to successfully become a data-driven organization
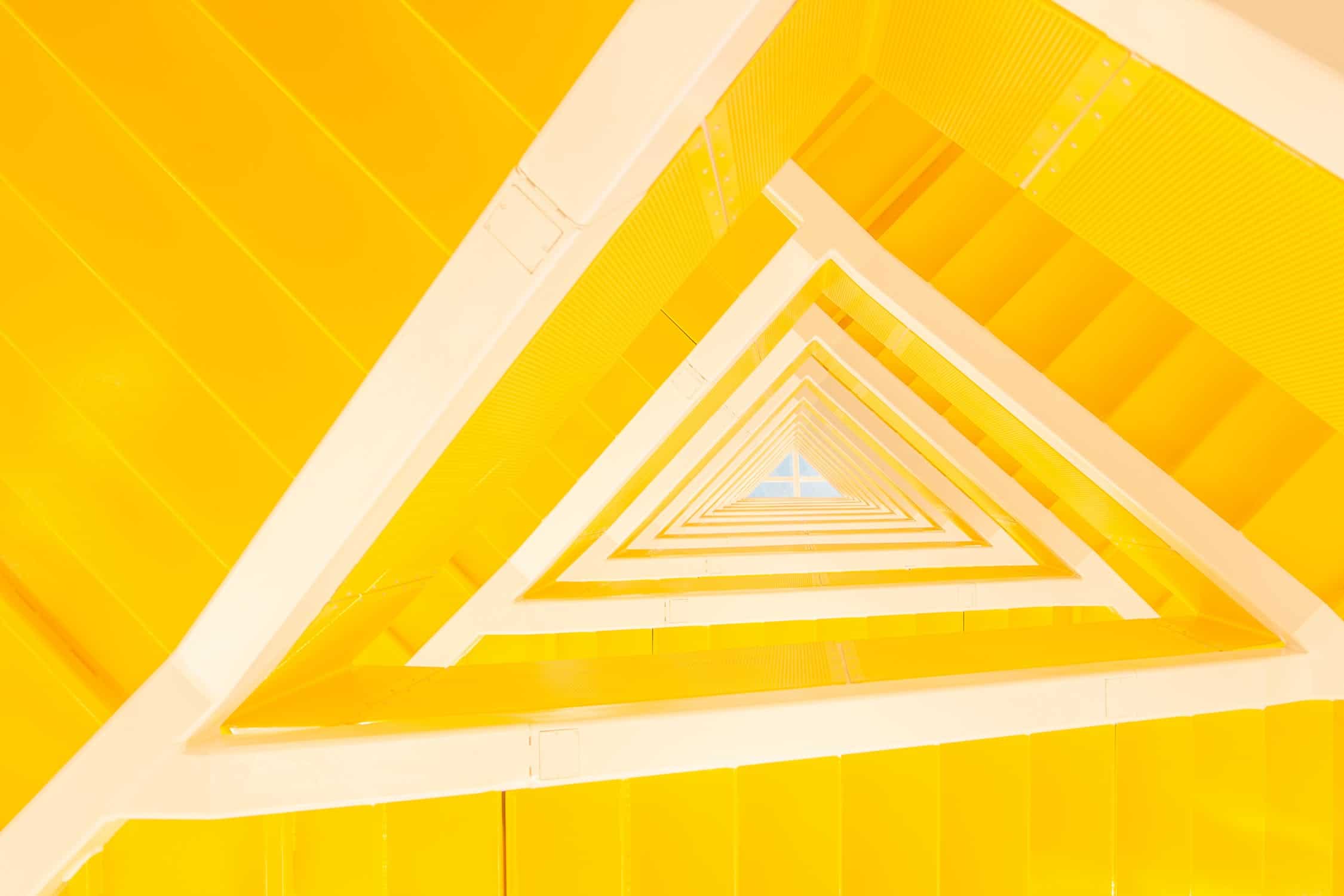
McKinsey believes that by 2025 most companies will be using data to optimize their operations and empower better decision-making. We outline the consultancy's seven key characteristics of data-driven enterprises and how businesses can achieve them.
Organizations today understand the importance of data to both delivering operational excellence and meeting longer-term challenges, such as improving customer experience, digital transformation or becoming more sustainable.
Many have started on their journey to create data-driven enterprises. In fact, McKinsey believes that by 2025 most companies will be using data to optimize their operations and empower better decision-making. In a recent report the consultancy outlined the seven key characteristics of successful data-driven enterprises and how to put them in place:
1. Data is embedded in every decision, interaction, and process
Currently many organizations have launched initiatives to increase data use, but these are often sporadic. They might be limited to specific departments or use cases and are not rolled out holistically across the entire organization.
The challenge is to scale these pockets of success to make data part of every employee’s daily life and central to company culture. Often data sharing is held back as information is stored in different silos and is only accessible and understandable by experts. What is needed is to democratize data, making it simple and straightforward for every employee to access and use the data they need, without requiring expert help, additional skills or training.
This shift has to be founded on a data-driven culture, where everyone is data literate and can find the data they need through self-service. The result is faster, more informed decisions, better understanding of performance and increased efficiency as traditional approaches are replaced and automated.
2. Data is processed and delivered in real-time
A second challenge many organizations face is the time lag between data being created and when it is available for sharing and reuse. This is becoming even more of an issue as businesses increasingly rely on data from different sources, such as Internet of Things sensors inside and outside their organization. What is needed is a flexible data architecture that can bring all this data together so that it can be understood and acted upon by all.
For example, the city of Orléans’s open data portal brings together datasets, including air quality information from a network of sensors, so that citizens have an up-to-date picture of pollution in different parts of the city. Orléans also shares data in innovative ways, including providing QR codes in its public parks that visitors can use to find out more about particular plants.
3. Flexible data stores enable integrated, ready-to-use data
The data volumes that organizations generate are growing exponentially, but in many cases, it is difficult to bring this information together and make it easily available to employees. Businesses need to centralize data, organizing it via a portal that can be accessed by anyone within the company. They should give users the tools they need to understand data, whatever their skill levels, creating data experiences that display it as maps, graphics, charts and other data visualizations.
Businesses should start by talking to users, from across the organization, in order to identify the critical datasets that they require. These can then be brought together to add value and create new data services and products, such as by combining different information about customers to create a single, complete view of their behavior.
4. The data operating model treats data like a product
Data must underpin every part of the organization and be available to all. However, often it has no true owner, meaning no one person or department is responsible for it. In the data-driven enterprise there are dedicated teams to manage data and ensure it is always up-to-date and meets standards and regulations, such as around confidentiality and anonymity.
This data is then made available to all, along with easy-to-use analytics tools so that users can self-serve and find the data they need. These data products not only help drive greater efficiency and innovation internally, but can also be provided externally to partners, potentially creating new revenue streams.
5. The Chief Data Officer’s role is expanded to generate value
Currently many Chief Data Officers are essentially responsible for compliance, policing data collection and use so that it meets regulatory guidelines and company policies. They need to move from being a cost center to a profit center, encouraging the greater use of data within the organization, driving greater data literacy and collaborating with business units to understand and meet their needs.
There are multiple ways of organizing data teams in organizations. Our own research found that respondents who have not yet launched data projects are more likely to pick a centralized structure. This allows organizations that are just getting started to centralize knowledge and involves fewer resources than if they were to set up cross-functional teams.
As they mature, businesses can then switch to cross-functional teams, with experts integrated into each team. This leads to better communication, alignment with a single global data strategy, the development of an internal data culture and the sharing of internally acquired knowledge.
6. Data-ecosystem memberships are the norm
We are now in a data-driven world – meaning the possibilities of information sharing and reuse stretch far beyond individual organizations. That means collaborating with ecosystem partners and other stakeholders is vital to maximize the value of data. Information can be automatically supplied in formats that are immediately re-usable or combined with other data sources to increase its value.
For example, telecoms company SFR automatically collects anonymized SIM card data as its customers move around. It is now using this data to provide insights into people’s movement and behavior, creating a new service: SFR Geostatistics. This opens up a new revenue stream for the company, as other organizations can buy data or expertise, and use this for planning new projects.
Digital energy solution specialist Schneider Electric has also made its data available widely to its ecosystem of partners. Through its Exchange portal, users can learn about digital products and service providers, or they can even discuss common issues with their peers through open communities.
7. Data management is prioritized and automated for privacy, security, and resiliency
Clearly any internal or external data sharing must meet compliance requirements, particularly when it involves customer information. However, protecting confidentiality and security does not have to be a time-consuming manual process. It can be automated following specific data ethics frameworks and processes.
For example, UK Power Networks runs a data triage process on every dataset before it is shared. Data is evaluated based on multiple criteria (such as privacy, security, ethics, intellectual property, data quality) before deciding whether it can be published or if it needs further work to anonymize it. As part of its commitment to transparency UKPN is sharing its data triage methods so they can be used across the sector.
Whatever sector an organization is in, successfully harnessing and sharing data provides the opportunity to create value, make better decisions, increase efficiency and collaboration and launch new services. With 2025 coming up fast, now is the time to focus on becoming data-driven for the future.
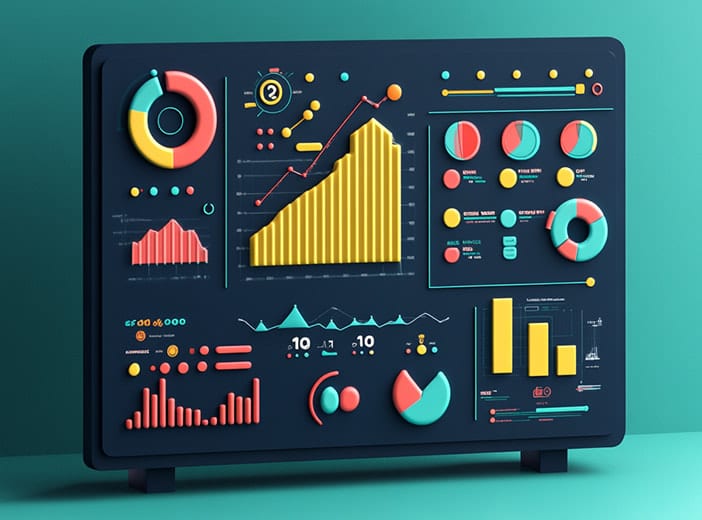
In an increasingly data-driven world, understanding the differences between data, metadata, data assets, and data products is essential to maximizing their potential. This is because these interrelated yet distinct concepts each play a key role in driving digital transformation by facilitating data sharing and consumption at scale.