Data governance vs data management: what are the main differences?
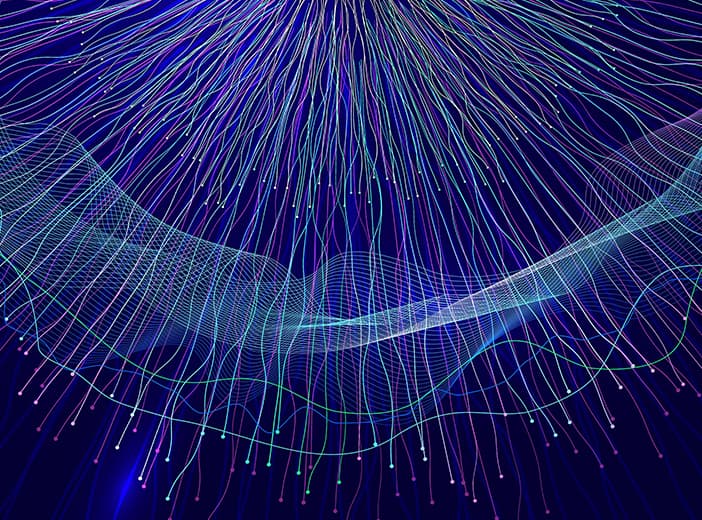
To maximize the value of their data, organizations need to focus on both data governance and data management. What are the differences between them and how do they work together? Our blog explains more.
Organizations today understand the importance of data. They are focused on ensuring that it is available and accessible at scale both internally and within their wider ecosystem in formats that everyone can easily understand and consume.
Turning data into value requires a combination of processes, technology, and culture in order to securely connect the right people with the right information to increase efficiency, save time, make better decisions and ensure transparency and compliance. Delivering this seamless experience involves both data governance and data management. This blog explains how they work together, the differences between them, and the role of data portals in achieving successful data governance and data management.
What is data governance?
Businesses are now generating and collecting huge volumes of data. Whether from business systems, Internet of Things (IoT) sensor networks, from partners or publicly-available sources, data is a key asset that needs to be shared across the organization and its ecosystem. It enables better decision-making, improved efficiency, and greater transparency around operations and performance.
However, the sheer scale of data volumes, and the variety of sources they come from complicates successful data sharing. Information can remain trapped in departmental silos, be in the wrong formats, be difficult to understand or may be poor quality. All information has to be kept secure and protected, especially sensitive data (such as personally identifiable information) which needs to only be available to authorized people to ensure compliance with regulations such as the GDPR.
This is where data governance comes in. It sets the rules and processes for how you handle and use the data collected and used within your organization and beyond. Essentially, it both manages risk around your data and enables it to be utilized in ways that benefit the business.
What are the goals of data governance?
Data governance meets both reactive and proactive goals.
Reactively, data governance ensures that:
- Data is high-quality, and does not have gaps or inaccuracies
- How data is described and used is consistent across the organization. For example, the same formats and descriptions are used for data fields (such as customer names) in different departments
- Data is trustworthy, meaning it is accurate, timely, reliable and that it is clear what it covers
- Data is kept secure, being protected from hacking and theft, while only being accessible by authorized people within the organization
- Data is used correctly, and is not being misused by employees or technology systems such as AI models
- Data remains private, protecting the privacy and rights of individuals
- Data is compliant with applicable regulations (such as the GDPR or sector-specific legislation, such as in financial services) and complies with internal rules and good practice
Proactively data governance delivers value:
- Breaking down silos between teams, and increasing collaboration through data
- Boosting innovation, efficiency, and agility by data sharing across the organization and its ecosystem
Helping create a data-driven culture, thus enabling data democratization
How do you structure data governance programs?
Data governance programs normally contain:
- A clear framework that sets out the standards and processes around managing data, including how it is stored and protected, and who is responsible for the upkeep of specific datasets
- A common language and terminology used to describe data across the business to ensure consistency
- Monitoring and management processes to ensure that data governance standards are being met
As data governance spans the entire organization, it requires the input and buy-in of all departments. While headed by the Chief Data Officer, the data governance team should involve data owners and data stewards from across the business, along with HR and communications to ensure its benefits are clearly shared with all and that everyone understands the importance of following it.
What is data management?
Data management covers the end-to-end process of collecting, processing, storing, sharing and using data across an organization and its ecosystem. Essentially it handles the technical challenges of handling all of an organization’s data and making it available, such as integrating different systems, reformatting data to ensure consistency and sharing it with users.
Organizations use a wide range of tools as part of their data management stack:
- Data creation: systems creating data, such as CRM solutions for customer data management, business software, or external sources such as social media
- Data collection: solutions that bring data together to make them easier to analyze. These include data warehouses, data lakes and data lakehouses
- Data management platforms (DMPs): This is a software platform used to collect and manage internal and external data in order to target customers.
- Business intelligence (BI)/data visualization tools: Once data has been prepared data analysts use business intelligence tools to run reports and queries on it, or harness data visualization software to turn tabular data into maps, dashboards and other graphical forms.
Given the growth in data volumes, the data management stack has to be able to scale effectively to handle both the amount of data and the complexity of the data landscape. Data management needs to ensure that data flows seamlessly across the business and its ecosystem, empowering users with real-time access to reliable, up-to-date information.
What are the main differences between data governance and data management?
As can be seen, data governance and data management are closely interconnected and you need both within your data strategy. A good analogy is building a house. You need the plans for how the house should be built (data governance), which are then used to actually construct it (data management). Building a house without a blueprint risks major issues – equally, plans are not enough if they remain on paper. There are four main differences between the two disciplines:
Strategy vs implementation
Data governance sets the overall strategy and processes for how data is used within the organization. By contrast, data management focuses on the technical solutions and actions needed to deliver on this strategy.
Different teams
Data governance programs are headed by the Chief Data Officer or equivalent, working with business leaders. As a more technical domain, data management normally falls under the control of the IT team, albeit with input from data leaders.
Different metrics
The success of data management activities is measured through technical metrics – such as data availability and speed of throughput. Data governance is judged on higher level KPIs, such as increasing data usage, creating value from data, and ensuring compliance.
Different tools
The data management stack covers the technical tools needed to collect, process, enrich and make data available. Data governance software focuses more on monitoring to ensure quality standards and processes are met, and creating a common vocabulary for how data is described across the business.
What is the role of data portals in delivering successful data governance and data management?
As we’ve established, organizations need effective data governance and data management to industrialize their use of data and maximize its value. Data portals provide a central space to make data available across organizations, ecosystems or to the wider world. They provide a focal point for both data governance and data management:
Ensuring quality
To upload data assets to a portal, they have to undergo a range of data governance processes to ensure quality, accuracy and timeliness. These mean that data assets on the portal can be used with confidence, as unreliable data is weeded out and all formats are standardized.
Delivering data to users
Data portals make all data assets available in understandable, accessible formats, such as through visualizations and dashboards, so that they can be discovered and used by everyone. This proves the value of data, and acts as the final point of the data management journey, justifying investment in tools and delivering ROI. Data portals also use connectors and APIs to enforce data governance standards up and downstream in data flows.
Enforcing security and compliance
Data portals feature access and rights management capabilities that ensure that data is protected and is only available to authorized users. This supports compliance with data governance rules and delivers an audit trail for management.
Scalable to meet growing needs
Data volumes are continually increasing, which can put traditional data management infrastructures under strain. As data portals are SaaS-based they scale to meet the organization’s needs, ensuring data availability no matter how many users or data assets are on the portal.
To maximize value from their data, organizations need to master both data governance and data management, putting in place robust policies and processes, backed by scalable technology and tools. Data portals support both effective data governance and data management, making them an essential part of your wider data strategy.
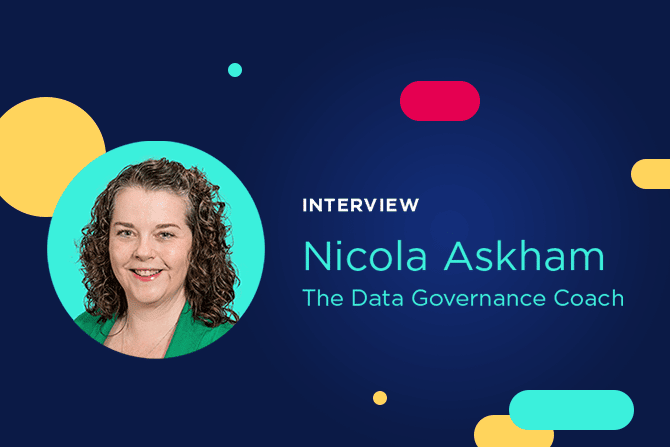
What are the practical steps that organizations need to take when it comes to data governance? How can they ensure their programs deliver real business benefits? To learn the secrets of data governance success we interviewed industry expert Nicola Askham.
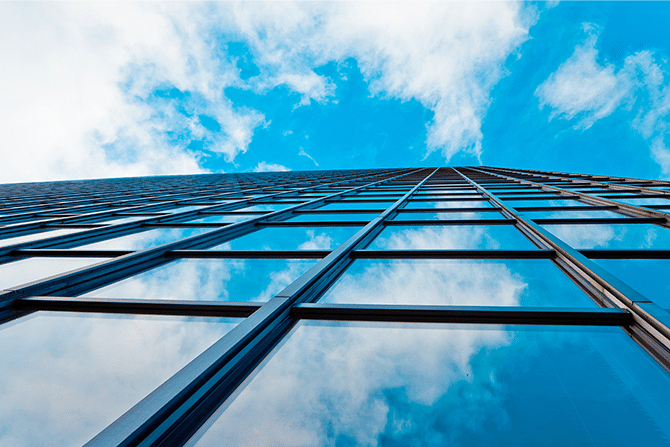
Why do many companies fail to effectively democratize data access? According to new insights from McKinsey it could be due to an overly narrow focus on use cases at the expense of building a scalable data value chain. Our blog explains the key components you need to industrialize data use and unlock greater value.
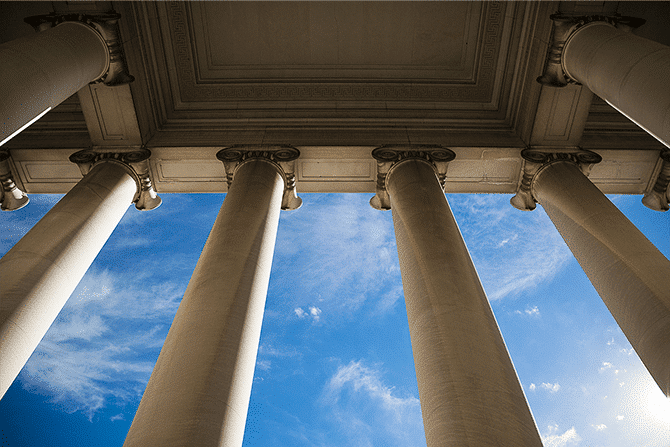
What are the challenges municipalities face when it comes to effective data governance. We look at the importance of data portals and robust data governance programs to enable municipalities to securely share reliable, compliant data internally and externally.