Data lake or data warehouse: which is the best option to deliver value from your data?
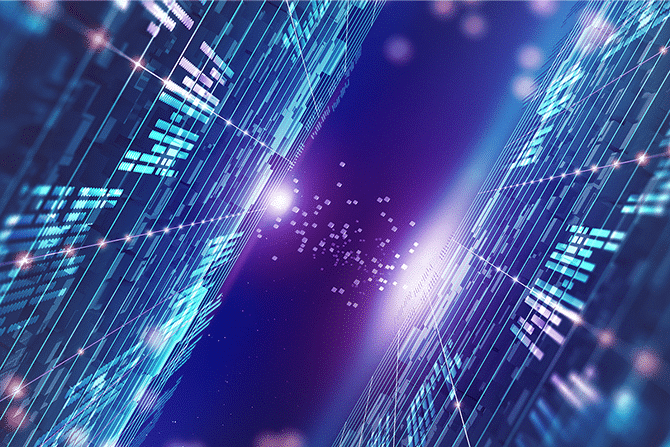
This article outlines the data lake and data warehouse storage models, explaining their advantages and disadvantages for your organization.
Organizations are experiencing exponential data growth. This brings a major challenge around how you choose the right structure to organize and store your information. This strategic choice can have a major impact on data quality and security, but can also limit how you can democratize data and use it effectively. This article outlines the data lake and data warehouse storage models, explaining their advantages and disadvantages for your organization.
The data lake to store large volumes of data
With the explosion of data volumes and the rise of Big Data, organizations need to be able to effectively store and work with this information. This is where the data lake model comes in.
What is a data lake?
A data lake is a storage space that contains all of an organization’s data in a raw form, such as emails, PDF files, tables, images, and videos. It can contain structured, semi-structured, or unstructured data.
In this model, data is stored generally, rather than being optimized for a specific purpose. It can be accessed for a variety of immediate or future uses, depending on the organization’s needs.
How is a data lake designed?
The data lake’s architecture is designed to be unconstrained in terms of size, type of data within it or its structure, with data able to be stored in the cloud or on premise).
There are major differences between how data is added to a data lake and a data warehouse. A data lake follows an ELT (extract, load, transform) model. This means that organizations retrieve data from different data sources and load it into the data lake in its original format. Only then do they transform and process the stored data to meet their specific needs. Achieving this requires data experts to use a multitude of specific tools for data ingestion, resource allocation, content indexing, restitution, graphics, migration, and analysis.
What are the best uses of the data lake model?
The storage capacity of the data lake allows organizations to access all types of information in real time. As data is easily available in its raw form, data scientists can easily extract it for specific uses. Moreover, unprocessed data can be used in machine learning, since algorithms need as much data as possible to learn more efficiently. However, to deliver its benefits the data lake model requires advanced data expertise and skills.
Finally, because of its simplicity of management, the data lake has lower running costs. This is especially true for cloud data lakes, which are generally less expensive than on-premise implementations.
The data warehouse, the traditional data storage model
What is a data warehouse?
A data warehouse is a place where data is stored. It appeared in the 1990s and is one of the first ways of centralizing operational data. As such, it only stores structured data, i.e. information that has been previously filtered, cleaned and processed for a specific purpose. This is often for commercial and marketing analysis, and for business intelligence across the organization.
By using a data warehouse, companies are able to run specific analyses around predefined use cases.
How does a data warehouse work?
As it only works with structured data, the architecture of the data warehouse is defined well before information is stored. Creating a data warehouse therefore requires time, advanced data expertise, and above all a significant cost.
And these requirements also continue when it comes to data management. Rather than the data lake’s Extract, Load, Transform (ELT) model, adding information to the data warehouse requires companies to follow ETL (Extract, Transform, Load) principles. This means data must be extracted from its original source, transformed/cleaned and imported. This approach is more time-consuming than ELT.
To simplify data management, it is also possible to group information in data marts within the data warehouse. These are subsets of data focused on a theme or business department.
It is only at the end of the ETL process that the stored information can be used and analyzed by data analysts or data scientists.
What are the best uses of the data warehouse model?
Unlike a data lake, a data warehouse allows you to:
- Prioritize data: data warehouses only store information that is operationally useful for the organization.
- Guarantee data consistency and quality: as data is processed and cleansed, users can use the available information with confidence.
- Reduce storage space: By limiting the amount of data stored, data warehouses do not waste space on unnecessary or obsolete information.
However, data warehouses also have a major limitation – it is complex to set up and integrate new data.
While the ultimate goal of a data warehouse is to help teams identify key performance indicators and make better decisions, people can’t leverage the data without expert intervention from data analysts. With a data warehouse, data is not really democratized throughout the organization, since not all employees can easily access or manipulate it.
Additionally, not all companies possess the internal skills to design the architecture of the data warehouse, nor to feed it regularly by integrating only filtered, cleaned and processed data. Between construction, data transformation, analysis, maintenance and team training, the cost of a data warehouse can amount to several hundred thousand dollars for organizations. The cost/performance balance must therefore be taken into consideration when opting for this solution.
Beyond the cost, the data warehouse has serious limitations in terms of usage. Even if it allows data transformation, the models it works with are still very complex and can only be mastered by specialists. That prevents data being used to benefit the entire organization.
What are the limitations of data lakes and data warehouses?
Both the data lake and data warehouse models have certain limitations as centralized storage solutions:
- The volume of data: users of a data lake do have access to large amounts of raw data. However, this is difficult to exploit in the absence of clear data governance. Very often, finding the right data in a data lake architecture is like looking for a needle in a haystack.
- Cost: data lakes and data warehouses require significant financial investments from organizations, both to set-up and to run.
- Users: data warehouses or data lakes are mainly used by skilled data analysts, data scientists or other experts. They have a thorough knowledge of the organization’s information systems, and above all, they are able to use specialist data analysis tools. This allows them to find useful data, exploit it and analyze it. This is not possible for other, non-expert members of staff.
- Uses: as you need to be an expert to handle data lakes and data warehouses, the uses of these solutions are clearly limited. In both cases data is difficult to understand and interpret by non-expert employees, as it is not available in formats that meet their specific needs. To get full value from data, every user should be able to consume all types of data through visualizations that allow them to understand information and use it to make more informed decisions and use it in new ways.
Whether it’s a data warehouse or a data lake, these two data storage solutions have limitations in terms of flexibility, usage and accessibility. To gain maximum value from data and drive data democratization, you need to make it available and understandable by all. This increases competitive advantage, reduces costs, and enables the creation of new services, for example. If it isn’t being used, then data has little value and is difficult to monetize. Fortunately, with Opendatasoft, you can make your data more accessible and reusable by everyone.
Get your data out of the data lake and data warehouse to drive data democratization
Our latest study with Odoxa reveals that only 31% of decision-makers say they have the necessary resources in terms of personnel, tools and governance strategy to make data widely accessible and encourage its use.
Why is it that despite the deployment of numerous solutions such as data lakes and data warehouses, data is not sufficiently valued? What is the missing key to unlock the potential of your data and make the investments you have already made profitable?
With an architecture based solely on a data lake or a data warehouse, business units are dependent on data specialists to be able to access the information they need, cross-reference it with other data or create data visualizations and dashboards. Because of this, some organizations have decided not to invest in data strategies because they lack the resources or specialist skills required to exploit data effectively.
However, in every sector data delivers competitive advantage. The knowledge that data contains must therefore be available internally to all employees, and also to external stakeholders. This will help transform corporate culture to create a data-centric organization with better decision-making, greater agility and higher efficiency.
So how can we really democratize data to serve the common good and meet today’s business and societal challenges?
A data experience platform to provide a single point of access to data for all your stakeholders
To democratize data in your ecosystem, you must meet certain key criteria:
- Data must be accessible by all stakeholders, based on their roles, via a single access point. Search and filtering capabilities are essential for your portal to allow data to be found in a few clicks.
- Data must be presented in formats that meet the different data needs of employees: such as through data visualizations, dashboards, graphs, or in raw formats.
- Data must be documented so that it can be understood by all and reused with confidence, particularly through metadata that is based on standards such as DCAT, DCAT-AP, and INSPIRE.
- Data must integrate into the normal business tools that employees use
- Data must be able to be enriched and formatted without advanced skills, thanks to processors that perform predefined actions. For example, processors can correct text and formatting, add geographic reference data, normalize a date or anonymize data.
- Data should be reusable through export options (Excel, CSV, API), and as GIS data, such as GPX for smartphone mapping. Formats should be compatible with national and international standards, allowing easy integration with national portals.
What are the uses of a data experience platform?
A data experience platform such as Opendatasoft allows you to create real business uses from your data, including:
- Creating a self-service data portal within your organization that allows all employees to easily access fact-based information and use it in their daily activities
- Creating data services to boost your efficiency, attractiveness and competitiveness
- Publishing open data portals to communicate transparently with as many people as possible.
Without a data democratization platform, creating these use cases would take several months or even years.
Our customers clearly benefit from time savings through using our platform, which allows them to get up and running very quickly:
- Schneider Electric connects its cloud and data lakehouse solutions, including Microsoft Azure and Databricks, to our platform to quickly feed its data marketplace created with Opendatasoft.
- ICF Habitat, a subsidiary of the SNCF group, is able to create internal use cases around data in a few months. Previously it took years when the teams had to go through IT.
- The financial director of insurer Lamie Mutuelle estimates that they have personally saved about 3 days per month thanks to the adoption of Opendatasoft within the company. The platform also increases the efficiency and reliability of the insurer’s operations by ensuring that data is always up-to-date and standardized.
Essentially, storage solutions such as data lakes and data warehouses are not enough to create value and innovative uses of data within an organization. The reason is simple: you need to bring data closer to the business and the different stakeholders in the ecosystem, democratizing it to drive data-centricity for all.
Learn more about the benefits of a data experience platform and our customers’ favorite features.
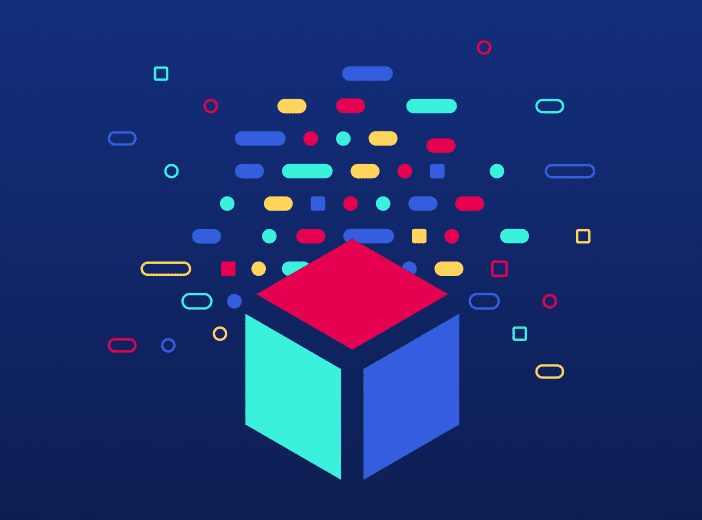
It can be hard to understand exactly what a data product is, given the many ways that the term is defined and applied. To provide clarity this article provides a business-focused definition of a data product, centered on how it makes data accessible and usable by the wider organization, while creating long-term business value.