Data, metadata, data assets, data products: understanding the differences between these key concepts
In an increasingly data-driven world, understanding the differences between data, metadata, data assets, and data products is essential to maximizing their potential. This is because these interrelated yet distinct concepts each play a key role in driving digital transformation by facilitating data sharing and consumption at scale.
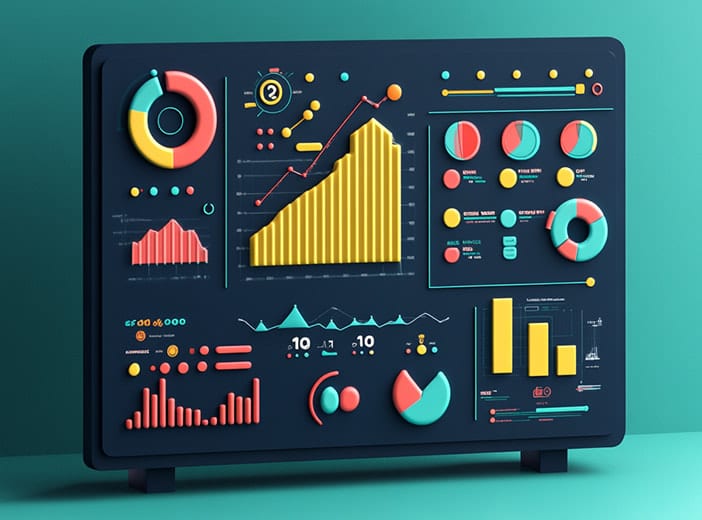
At a time where data volumes are exploding, it is increasingly vital for companies to understand and master the related concepts of data, metadata, data assets, and data products. These elements are much more than just technical ideas, and are in fact key approaches to creating business value. When properly understood, they not only improve decision-making and optimize business processes, but also ensure effective, ongoing data governance across the organization. This blog takes an in-depth look at these four concepts, explaining what they are, how they differ, and how they work together.
What is data?
Data refers to raw, untransformed information that is collected from multiple sources such as user interactions, Internet of Things (IoT) sensors, databases or CRM applications. This data can be qualitative (such as customer reviews) or quantitative (for example, sales figures or physical metrics).
Why is data important?
Data is the foundation of all analytics and is central to taking better-informed, more strategic decisions. It helps spot trends, improve performance, and personalize the user experience. Data is essential for three key reasons:
- Providing the basis for analysis and transformation: Raw data is the source material from which relevant insights are extracted. It therefore powers AI algorithms, analytics, and visualizations, making it possible to then make better-informed decisions and implement effective, data-driven strategies.
- Delivering a source of objective truth: As it hasn’t been processed or changed in any way, raw data provides a true, objective picture of what is happening. This means that it can be relied upon for creating bias-free analyses, delivering more accurate and useful insights.
- Powering diverse applications: Raw data is highly flexible and versatile. It can be adapted or enriched to meet a variety of needs, whether analysis, developing AI predictive models, or creating innovative solutions such as data products.
However, the usefulness of data depends on its quality, reliability and the ability to access it easily. On its own, isolated data is of little value. It only delivers benefits when it is used and shared effectively.
Data – a concrete example
Computer network monitoring is a good example of raw data. Sensors collect this raw, performance data on a continuous basis, without any analysis taking place. The data shows specific, unprocessed information, such as response time, CPU usage, and memory consumption metrics, but does not yet draw any conclusions or provide insights. To be useful, this data must then be analyzed to detect anomalies, predict outages, or optimize network performance.
What is metadata?
Metadata is data that describes data. It provides context to raw data to make it understandable, searchable, and actionable. By providing information such as the source, format, or structure of data, metadata makes it easier to manage, search for and use data within an organization.
Why is metadata important?
Metadata is essential for effective data management, governance, and usage at scale. It provides structure and context that maximizes data’s value while making it easy to understand and consume. Metadata delivers five key benefits:
- Data search and discoverability: Metadata acts as a compass for navigating a complex data environment. When data is well-described and organized using metadata, users can quickly locate the relevant information they need. This reduces the time spent on searching and increases the chances that the right data will be used by the right people in a timely manner.
- Data governance: Metadata is at the heart of strong governance, allowing comprehensive management, monitoring and control of all of an organization’s data. It provides transparency over where data is located and who has access to it, ensuring traceability while maintaining security.
- Quality and compliance: By describing the origins, transformations, and attributes of data, metadata helps ensure its quality and integrity. It also plays a crucial role in ensuring that an organization’s data complies with relevant regulations, such as the GDPR and CCPA, and that it is used ethically and responsibly.
- Data interoperability: Metadata facilitates communication between different systems, applications, and databases by standardizing how data is described and categorized. This ensures that data can flow seamlessly between different IT systems and teams.
- Collaboration: Metadata provides a common language for all stakeholders to describe data. This promotes mutual understanding and strengthens collaboration between teams, even those in different departments and roles.
Metadata – a concrete example
A document management system may hold thousands of different documents, created by a range of authors. Metadata provides a means to easily navigate all of this information. For example, it makes it possible for anyone to quickly search for, and find, a specific contract based on its author or when it was signed, saving time and facilitating better data access.
Learn more in this article: Metadata management: increase efficiency with Opendatasoft’s customized templates
What is a data asset?
A data asset is a digital object or entity composed of raw data that has been transformed and prepared to deliver business value. Unlike raw data, a data asset has been structured and enriched to make it reusable and consumable by employees within the business. For example, raw data can be transformed into a report, or it can be described and put into a standard format (such as a CSV spreadsheet file, API or interactive visualization), allowing it to be accessed and reused widely.
A data asset is designed to be easily understandable and usable, whether by humans or by IT systems. It can come in different forms, such as a dataset, a report, a visualization, or a data service, and often includes tools to make it easier to access and use. Data assets can be structured (such as databases) or unstructured (documents, images). They can be used internally, shared for free with partners, made accessible in the form of open data, or even turned into data products, which we’ll talk more about later in this article.
Key characteristics of data assets
A data asset has four key characteristics:
- Usefulness: Every data asset should deliver tangible business value, meeting the needs of one or more user groups within an organization.
- Accessibility: Data assets should be easily accessible by authorized stakeholders, whether openly or through a simple and intuitive request for access.
- Documentation: Each data asset should be accompanied by clear, complete, and detailed metadata to make it easy to understand and use.
- Quality: The data used within a data asset must be accurate, complete, and reliable.
Why are data assets important?
Data assets play a key role in helping everyone use information within an organization, and therefore drive data democratization. They make data accessible and actionable in different forms, such as datasets, dashboards, or apps, thus maximizing its use by all.
Centralized, efficient management of data assets makes it easy to analyze, share, and reuse data. This leads to better-informed decisions based on reliable data, reducing risk while improving operational efficiency.
In addition, well-structured data assets strengthen collaboration between different departments by establishing a common language around data. They are a crucial step in the data value chain, by making information understandable and usable by everyone.
In short, data assets allow organizations to transform their raw data into a strategic resource, improving performance, decision-making and innovation.
Data assets – a concrete example
A dashboard that shows real-time data is a specific example of a data asset. In this case, the dashboard collects and transforms raw data generated by various sources, such as systems or IoT sensors, providing it in a form that is easy for users to understand and access. A second example is when a dataset is enriched with additional information or has its schema and documentation improved, making it consumption-ready.
What is a data product?
A data product is a self-contained solution or service designed to enable the usage and analysis of data. It can take different forms: dashboards, recommendation systems, predictive applications or actionable APIs. Unlike raw data, data products are complete solutions, specifically tailored to the needs of end users.
A data product is a specific type of data asset. It differs from other data assets through its structured, self-contained, and ongoing capabilities. It combines data, metadata, semantic models, and ready-to-use templates to address a specific use case, such as data sharing, monetization, domain-specific analytics, or integration into applications.
The key characteristics of data products
A dataset alone is not a data product. When designing data products, you need to make sure that they meet these key characteristics:
- Strongly promoted to meet specific needs: data products stand out amongst other data assets as they are designed to meet a specific need and to deliver value. They often benefit from increased visibility in data marketplaces, increasing their attractiveness and accessibility.
- User accessibility: One of the essential characteristics of data products is that they must be easily accessible by their users. This includes clear access to the underlying data, ensuring smooth and intuitive usage.
- Always up-to-date and scalable: Data products must be regularly updated and designed to adapt to increases in volumes or user growth without affecting performance.
- Data contracts: A data product is based on specific agreements, called data contracts, that define what the product owner agrees to provide, as well as how the data will be used. This ensures transparency and reliability for users.
- Promotion via data marketplaces: Data products are often promoted in data marketplaces, dedicated platforms that facilitate their discovery, sharing and monetization.
- Associated with a quantifiable cost (for IT) and a measurable value (for the business): to guarantee its relevance, a data product must present a balance between the IT costs it requires for its development, maintenance, and infrastructure, with the tangible value it delivers for the business, measurable by concrete KPIs.
Why are data products important?
Data products play a key role in transforming data into concrete solutions that generate value for companies:
- High usage: Data products are often created to meet strategic needs and are therefore designed to be used at scale, with a particular focus on usability, understandable documentation and regular updating of information. This ensures their optimal utilization, as end-users trust in the quality, relevance and timeliness of the available data they are built on.
- Accelerate strategic decisions: Through data products such as interactive dashboards or apps, decision-makers have access to real-time insights to guide their choices in a faster, better-informed way..
Find out more in this article: 3 reasons why data marketplaces are the only solution to turn data into value
Data products – a concrete example
Within an organization, a data product could take the form of a centralized, constantly updated customer information application. This app, accessible to all employees, provides complete information on every customer, and therefore plays a strategic role in facilitating customer interactions, optimizing business processes and ensuring consistency across the organization. It requires rigorous management, strong promotion to drive usage and careful monitoring to maximize its effectiveness and added value.
The differences and interconnections between data, metadata, data assets, and data products
Data, metadata, data assets, and data products combine to create a powerful data value chain, maximizing the consumption and sharing of data at scale within organizations, while facilitating its democratization and providing seamless access for all stakeholders.
Data is the raw material: it is made up of facts, figures, events and unprocessed information. This raw data, whether qualitative or quantitative, provides the foundation on which data consumption is built. Raw data is essential because it captures real actions in their most basic, objective form, but it needs to be interpreted to unlock its full value.
Metadata, on the other hand, provides a structured framework around this data. It contextualizes, describes and organizes data to make it understandable and easily exploitable. Metadata delivers essential information such as the data source, its format, when it was collected, and the transformations it has undergone. Metadata therefore makes it easy to discover and manage data, ensuring compliance, while guaranteeing its transparency and traceability. Metadata makes it easier to locate relevant information, build trust in its reliability, and ensure its accessibility.
Data assets turn raw data into business value. They can come in a variety of forms, including datasets, visualizations, and apps. They are designed to be easily consumed and reused, often containing built-in tools that make them easy to use by non-technical staff. These assets can be structured, such as a database, or unstructured, such as documents or images. In all cases, they are created and shared with the aim of meeting specific needs and providing valuable insights for users.
Data products go one step further by transforming raw data and metadata into ready-to-use, actionable solutions. A data product is a high-value-added data asset designed to meet specific needs and is used by a wide range of users on an ongoing basis. It is managed strategically throughout its life cycle, to ensure it is high-quality, well-structured, regularly updated and well-used. Promoted through a data marketplace, it combines raw data and metadata in a structured way, enriched by analytical models and automated processes. By facilitating the direct use of data, a data product creates business value by responding specifically to the needs and expectations of end users.
Overall, data, metadata, data assets, and data products are not standalone concepts, but instead are interconnected elements that together enable companies to make the most of their information assets. Every component of this chain – from the collection of raw data to the creation of ready-to-use data products – plays a crucial role in an organization’s ability to fully leverage its data and extract strategic value from it.
Turning data into business advantage
Being able to understand and master the concepts of data, metadata, data assets and data products provides competitive advantage in an increasingly data-driven world. Together, they form an ecosystem that is essential to data democratization and turning information into value across the entire organization.
This means that companies need to invest in rigorous data management practices in order to efficiently and intelligently harness their growing data volumes. By focusing on each link in the data ecosystem and meeting their users’ expectations around data immediacy, accessibility, and self-service, organizations can meet their current and future business challenges and remain competitive in a constantly changing, transforming world.
In short, data is the basic material, metadata is the blueprint, data assets are data made intelligible and shareable, and data products are specific solutions designed to meet high-value business requirements. A data marketplace is essential to delivering all of these four concepts, providing a centralized space for collecting, governing, discovering and creating value from data across the business and beyond.