How do you structure your data team for success?
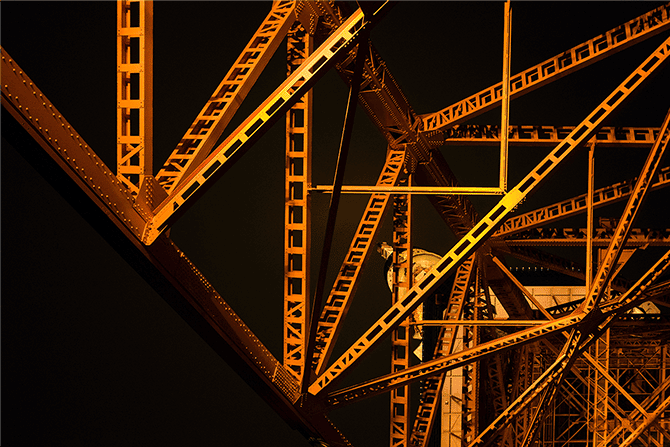
Ensuring data flows effectively around your organization means adopting the right structure for your data team. What are the options and when should you pick a centralized, rather than decentralized approach? Our blog explains what you need to know when structuring your team.
Organizations understand the increasing importance of data democratization and how it helps them become more agile, efficient, productive and innovative. However, given that companies now have access to ever-growing volumes of data, from more and more internal and external sources, how do they structure a data team to meet their needs?
There’s no silver bullet or perfect structure for building a data team. Instead you need to look at your specific business needs to choose the one that’s right for you, backed up with the right skills, culture and governance to drive results.
What are the different structures to choose between?
When building a data team, the main choice is between having a team that is centralized, decentralized or a hybrid of the two. So what does each approach deliver, and what are their particular advantages?
Centralized data team model
Everyone involved with data, from data analysts and data engineers, all the way up to the Chief Data Officer (CDO), works in a single, central team. This manages data for the entire organization, and is responsible for sharing it with the business, providing reports to management and ensuring consistency and good governance. Essentially it acts as a supplier or consultant to the organization, handling everything involved with data.
Advantages:
- Focus: All data projects are run and governed centrally, meaning the CDO can prioritize resources to ensure they are applied in the right place to meet the organization’s needs.
- Skills and learning: Team members can learn from each other and develop their skills, whether they are data analysts or data engineers.
- Retention and progression: As team members become more experienced, there are opportunities for promotion, helping keep them engaged and retaining their skills.
- Resources: Rather than different departments buying and maintaining data tools, everything is managed centrally. This brings down management, training and licensing costs.
- Governance: By centralizing data responsibilities, it is easier to ensure good governance, security and data compliance.
However, particularly as an organization’s needs grow, the central model can create challenges:
- Workload: The more organizations embrace data, the more work the central data team will need to carry out. This means they can become a bottleneck, slowing down data projects and limiting business support.
- Distractions: Again, as employees have more questions about data, this can overload the data team with queries and short-term projects, meaning vital longer-term activities are never progressed.
- Disconnect with business needs: As the data team is separate from business units, it can be difficult to get a deep understanding of business priorities and needs.
- Seen as the data police: With the central team responsible for areas such as compliance, governance and security, they may be seen by the rest of the business as solely policing data use, rather than adding value.
Decentralized data team model
At the opposite end of the spectrum, the decentralized (or embedded/cross-functional) data team model disperses data specialists across the business, embedding them within different business units. They report directly to business managers within their departments.
Advantages:
- Agility: As data people are closer to the actual creators and users of data they can act more quickly to meet their needs and priorities.
- Business-focused: Data teams have a greater business understanding and domain knowledge, allowing them to be more responsive to what each department wants.
- The right resources: Rather than ask a central data team for help, all the resources required are already within the decentralized team, allowing them to work faster.
Again, there are challenges that need to be met:
- Lack of consistency: There’s the potential for multiple projects to be run at the same time by different teams, risking data consistency, duplication, and preventing the creation of a single source of truth.
- Silos hit productivity: Each embedded data team tends to be smaller than under a centralized approach. That lack of scale can mean team members aren’t able to ask colleagues for help or develop their skills by learning from peers.
- Management issues: In a decentralized model, business people are ultimately managing data specialists. They are unlikely to have the technical background to understand their projects, what they need to be successful or how to keep them engaged.
Hybrid/federated or Center of Excellence data team model
Unsurprisingly, organizations want to gain the best of the centralized and decentralized models, without their drawbacks. That’s why many mature data-driven companies have evolved a third approach – adopting a hybrid/federated or Center of Excellence data team model. In this case, data people are embedded within business units, but there is also a central group that provides support, training and overarching data strategy. Taking this approach is a key part of the data mesh concept, which balances central and local control and involvement. You can read more about data mesh in our introductory blog.
Advantages:
- Balance: There’s a strong balance between the priorities of business units and the wider organization, with data leaders able to pick the most important projects to work on.
- Governance: All data is still governed centrally, ensuring consistency and security.
- Data culture: Employees see that there are data people all around them, helping develop a data culture across the organization.
- Resources and training: Through access to training, networking with their peers, and centralized resources, data staff are able to develop their skills and knowledge.
However, while the hybrid model delivers benefits it does add another layer of complexity to the structure, increasing costs and management time. It also means an organization will need to employ a significant number of data specialists, both centrally and within departments. All of this means it is best suited to larger organizations with a high level of data maturity – otherwise it can push up costs without delivering expected results.
How do you pick the right structure for your organization?
Before choosing the structure for your data team, organizations need to answer a number of questions:
- Size: How large are they and what resources do they have to dedicate to data projects?
- Maturity: How mature are they when it comes to using data across the organization, at both a business and technical level?
- Skills: What skills do they have within the business already and where are they located?
- Industry: Different sectors have different data needs – for example, regulated industries such as finance or healthcare have to meet additional compliance rules, impacting how they structure their team.
The model organizations adopt is likely to change over time as they become more mature. For example, research by Opendatasoft found that 41% of organizations who were still in the planning stage of their data projects had a centralized team, as this approach gave them the level of control required to launch initiatives. Just 32% had adopted a decentralized approach. By contrast, 45% of more mature organizations had decentralized their data team structures as they’d built up the skills, experience and governance to enable them to embed data specialists within departments.
Whatever approach you adopt it is vital to keep reviewing and monitoring it, with data leaders such as Chief Data Officers talking to senior management from other areas to ensure that the structure always remains fit for purpose and maximizes data democratization across the organization.
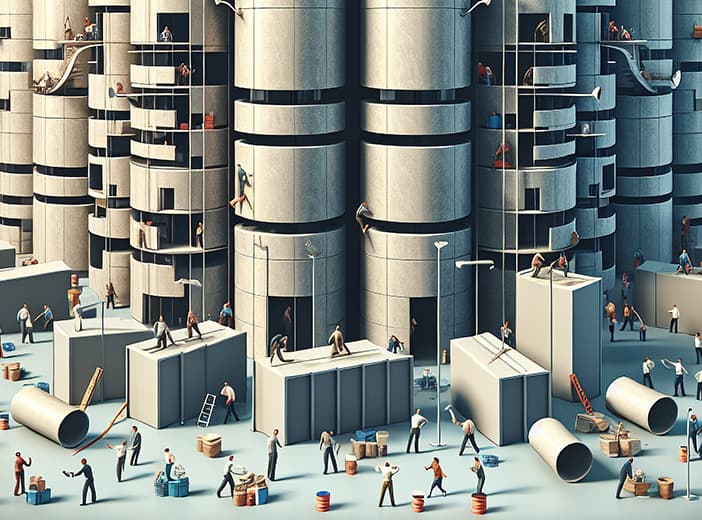
Organizational silos prevent data sharing and collaboration, increasing risk and reducing efficiency and innovation. How can companies remove them and ensure that data flows seamlessly around the organization so that it can be used by every employee?