The impact of GenAI on data management – predictions from Gartner
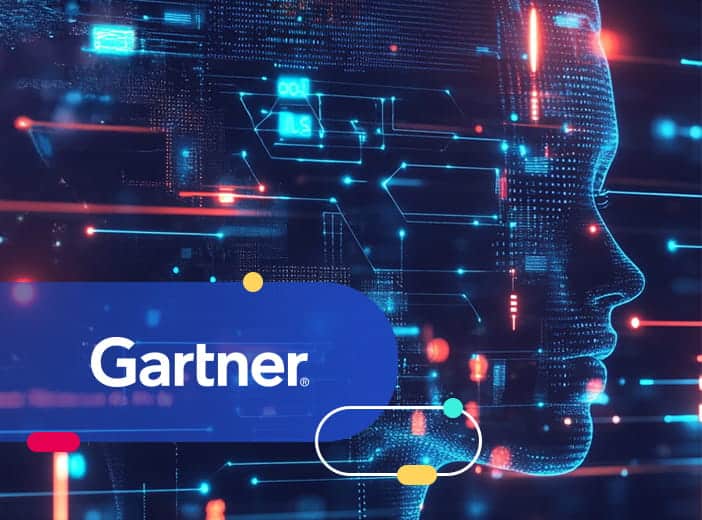
How can generative AI help Chief Data Officers and other data leaders to better manage their operations? Based on Gartner research, our blog outlines the key benefits AI can provide within the data management stack
Successful AI relies on high-quality reliable data, both to train Large Language Models (LLMs) and to underpin generative AI (GenAI) applications. However, AI can also play a major role in transforming the practice of data management within organizations, helping to improve productivity, efficiency, quality, and how data is used, impacting solutions, architectures, operating models, skills and use cases.
Looking forward, how can Chief Data Officers and other data leaders successfully deploy AI across their data management operations, solving pain-points and increasing data availability, such as through data marketplaces? A new report from Gartner explores the topic, and this blog shares some of its key conclusions.
The 4 key data management themes for 2025
In its report, Predicts 2025: 4 Ways AI Will Disrupt Data Management Markets and Solutions, Gartner outlines four key themes that are driving successful data management:
- Convergence – the need to reduce complexity in the data stack through closer integration of tools and ecosystems
- Democratization – meeting the last mile demands of users through seamless, self-service access to relevant data
- Augmentation – increasing the productivity of data teams, enabling them to successfully manage ever-growing data volumes and demands
- Cost optimization – better managing budgets and spend on solutions, especially those in the cloud
While these trends are key objectives for data and IT leaders, achieving them is complicated by:
- Increasing pressure from the business to manage and make available expanding numbers of data assets and data products
- Highly manual processes that see data management staff spending the majority of their time fixing issues and optimizing workloads, rather than on added value activities
- Escalating demand to develop AI applications for the business, within existing budgets and teams
However, as well as adding to workloads in terms of new projects, GenAI also provides an opportunity to transform data management by implementing it within the data stack itself. Gartner believes that “By 2027, AI assistants and AI-enhanced workflows incorporated in data integration tools will reduce manual intervention by 60% and enable self-service data management.“
The impact of GenAI on key data management themes
Looking at the four themes individually, Gartner outlines how GenAI can deliver benefits to each of them, something we have also seen in both our internal development and our work with clients using our data marketplace solution.
Convergence (of tools & technologies)
The past decade has seen an explosion in the number and range of separate tools used within the data stack, each focused on a different capability. This means that data teams have to spend time integrating solutions in order to build end-to-end data pipelines and ensure robust data governance. This adds to complexity, increases administration, and slows innovation. What is required is an ecosystem approach, where tools work together seamlessly, particularly by sharing metadata to ensure quality and governance. Data ecosystems are developing, such as those created by cloud service providers to speed up integration and reduce management overheads. GenAI within these ecosystems and platforms helps automate convergence and improves quality and productivity.
Democratization of self-service
Nearly half of respondents to the 2024 Gartner Evolution of Data Management as a Dedicated Function Survey highlighted self-service data preparation as a priority for investment. This is to meet the critical requirement to turn data into value by sharing it across the organization and externally with partners, through data marketplaces and as data products. This need is growing rapidly, adding to workloads on data teams to make data usable and understandable by their non-technical audiences. Deploying GenAI through LLMs helps bridge the gap between data and its use, such as by enabling the automated creation of visualizations, dashboards and other graphics, based on user prompts. These can be created more easily by those without technical skills, whether employees or data team members, helping democratize data while reducing pressure on resources.
Augmentation of data engineering tasks
Managing data stacks and ensuring data availability consumes a large proportion of data team time and resources. These administrative activities prevent teams focusing on more strategic, innovative work that would benefit the wider business. Deploying GenAI within data management helps automate many tasks, reducing the need for manual intervention and therefore freeing up time. For example, incorporating GenAI within data management tools can allow analysts to query and transform data through natural language, while end-to-end metadata management ensures consistency and governance. GenAI can also be used directly by data engineers to find answers to queries on how to optimize platforms, and to generate code based on prompts.
Cost optimization
While the cloud has brought major benefits in terms of speed of deployment, reliability and the removal of fixed infrastructure, it does need to be managed closely to optimize costs across the data management stack. To achieve this, many organizations are already deploying FinOps tools and practices – adding GenAI to these will make the management process simpler and easier. Rather than hunting through data to uncover opportunities to make savings, data teams can use AI to both discover and automate optimization. By involving AI’s predictive capabilities, organizations can plan future spend to ensure it is right-sized to their needs.
How to benefit from GenAI in data management
To help data leaders plan effectively and benefit from GenAI, Gartner outlines a checklist of actions to take when it comes to data management:
- Explore the adoption of data management ecosystems that bring together tools and capabilities to reduce complexity and management overhead. Often, these are delivered by cloud providers, combining best-of-breed solutions and using AI to drive automation, consistency and quality.
- Evaluate the use of GenAI-augmented data management tools to reduce the need for specialist data engineering skills when it comes to creating and managing, transforming and governing data.
- Ensure you invest in metadata management to ensure data quality across the organization, prior to deploying LLMs
- Educate data teams on the use of natural language capabilities to understand and manage cloud costs
- Explore how GenAI can enable less-technical users to manage and consume data, understanding the impact on the skills mix and your team and organizational structures
- Investigate Retrieval-Augmented Generation (RAG) AI technologies and how they will benefit the creation of GenAI-based business apps
The Opendatasoft perspective
At Opendatasoft we are committed to democratizing data, ensuring that organizations can share it effectively with all relevant users, and thus maximize its value. AI is already a core part of our data marketplace solution, helping drive the consumption and sharing of data in three key ways:
- AI vector search to provide more accurate, contextual results, rather than relying on keywords. This is particularly important for search queries from non-technical employees, as they are unlikely to know and use specific data terms or language
- Similar dataset recommendations, offering additional data sources and products to users, based on their queries and usage
- GenAI-created visualizations, generated automatically through natural language prompts
These features reduce the load on data teams while providing all users with seamless access to information. Moving forward, we’re focused on increasing the use of AI within our data marketplace solution, and within our wider development practices. Want to learn more? Contact us to arrange a demo of our AI capabilities.
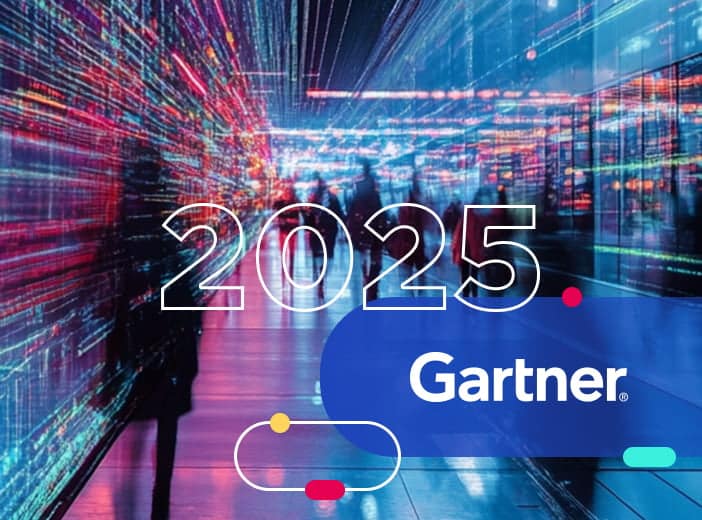
Growing data volumes, increasing complexity and pressure on budgets - just some of the trends that CDOs need to understand and act on. Based on Gartner research, we analyze CDO challenges and trends and explain how they can deliver greater business value from their initiatives.
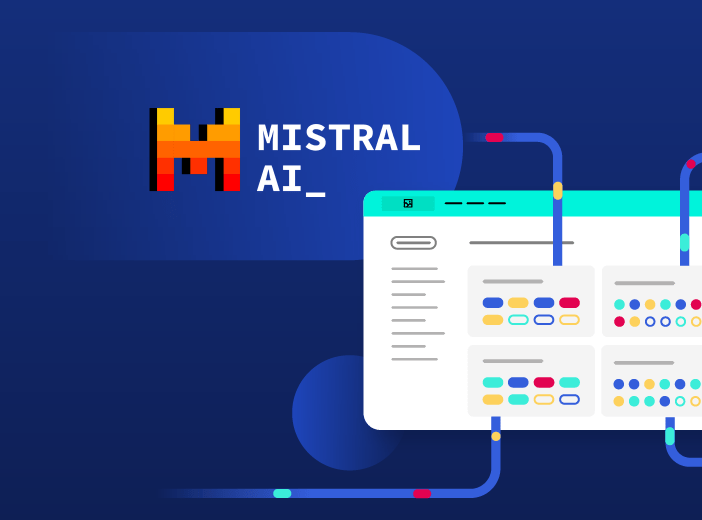
To give customers choice when it comes to AI, the Opendatasoft data portal solution now includes Mistral AI's generative AI, alongside its existing deployment of OpenAI's model. As we explain in this blog, this multi-model approach delivers significant advantages for clients, their users, our R&D teams and future innovation.
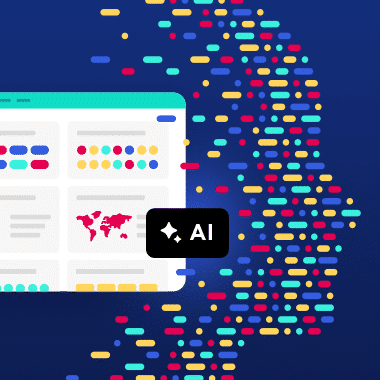
Over the past months Opendatasoft has been working to transform its data portal solution by enriching it with AI, helping clients to save time, improve the experience for their users, and reduce the risk of errors within processes.