The importance of data quality in turning information into value
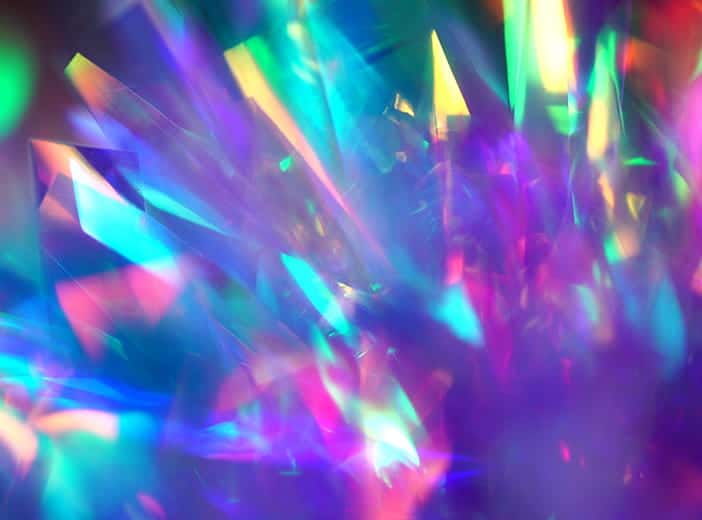
What is data quality and why is it important? We explain why data quality is central to building trust and increasing data use, and the processes and tools required to deliver consistent high-quality data across the organization.
Organizations are generating and collecting increasing volumes of data – but for this information to be used at scale, it has to be reliable, accurate, and trusted by employees and other stakeholders. Businesses therefore need to focus on data quality if they are to create real value from their information, drive greater usage, and support better decision-making. Otherwise poor data quality will add to costs, increase inefficiency, prevent collaboration and undermine security and compliance.
According to Gartner, every year poor data quality costs organizations an average of $12.9 million, holding back data sharing and preventing employees effectively using data in their working lives. What is data quality and how can it be improved?
Understanding the importance of data quality
High quality data shares multiple key attributes:
- It is accurate, without mistakes or errors
- It is reasonable, with data containing valid values within acceptable ranges
- It is timely, with information being up-to-date
- It is complete, without gaps or missing fields
- It is consistent, following the same formats and using the same units throughout the dataset, matching terms and definitions used across the organization
Reliable, high-quality data builds trust with users. This means they are confident about accessing and using it, as they are certain that it meets their requirements. Ensuring that data is trusted is particularly vital when scaling data use, such as through self-service data marketplaces, where data might be shared by another department and there is no existing relationship between the user and the data provider.
Poor data quality damages businesses in five key ways:
Poor decision-making
Inaccurate data leads to the wrong decisions being made, such as when creating strategies and tactical plans. For example, if sales figures seem to show that a particular product is both profitable and popular, businesses will make or stock more of it. If the figures are inaccurate, then this leads to the wrong supply chain and sales strategy, impacting revenues and profitability. The same applies if customer data is out-of-date, leading to missed opportunities to upsell or cross-sell products or services.
Lower efficiency
Manually fixing issues with low quality data takes an enormous amount of time within organizations. Often data analysts have to dedicate a significant part of their workload to checking, updating and cross-referencing information before they use or share it. This prevents them dedicating their time to higher-value activities that benefit the business. Equally, data can be used to automate previously manual processes – something that cannot happen if it is unreliable, out-of-date or in the wrong format.
Compliance and reputational damage
Under regulations such as the GDPR and CCPA organizations have a duty to safeguard confidential data and ensure it is accurate and reliable. Poor quality data, particularly if shared externally with regulators or partners, can damage reputations or lead to legal issues, triggering investigations by regulators and lawsuits from aggrieved consumers.
Inability to create new data services
Many organizations are harnessing the data they produce to create new data services that they can sell to existing and new customers. This will be impossible to achieve if data is unreliable or inaccurate, impacting new revenue streams and undermining existing customer relationships.
Holds back increased data use
Data should be central to how every employee works, helping them to be more productive, efficient and effective. However, if they do not trust or understand particular datasets or believe they are low quality, they simply will not use them. This prevents data democratization, collaboration between departments and digital transformation. Poor data quality is a particular issue if inaccurate information is used to train AI models, resulting in inaccurate outcomes and potential bias within results.
The challenges to delivering data quality
Given the business benefits of data quality, it seems obvious that every organization should be focused on ensuring their information is accurate, reliable and trustworthy. However, achieving data quality is held back by two major challenges:
Growing volumes of data
In an increasingly digital world, the sheer amount of information generated and collected by organizations is growing exponentially. Internal sources, such as business systems, customer databases and Internet of Things (IoT) sensors all produce enormous volumes of data, often created in real-time, while information collected or bought from external partners adds to the problem. Keeping track of all of these data assets, and ensuring they meet quality standards, is a major issue for organizations.
Data is scattered across the organization
These increasing volumes of data are being created and stored across the organization, such as in departmental systems. This makes building a comprehensive inventory of data a major task for data leaders and their teams. Differences between the terms used by different departments to describe data also leads to potential quality and trust issues. For example, the term “customer” might not mean the same thing when used in data generated by the sales or finance departments, leading to confusion and inaccuracies if information is shared or compared.
How to improve data quality
Improving data quality requires a strong combination of data governance processes, data quality tools, and training. There needs to be a comprehensive, end-to-end framework in place that covers all relevant data and builds trust with users so that they confidently use data in their working lives.
Data governance
Data quality begins with the creation and enforcement of robust data governance processes. Data governance covers how you identify, organize, handle, manage, and use data collected in your organization. It ensures data quality and compliance by setting out a framework for how data is stored, protected and shared, with data stewards appointed to look after individual datasets, and comprehensive monitoring to ensure data is used in accordance with corporate rules. As part of data governance it is recommended that organizations create a data dictionary, defining what terms used to describe data actually mean, in order to provide consistency across the organization.
Data governance is particularly important when data is being shared between departments or externally – users need to know what a dataset contains and who the owner is, so they can make contact with them if they have queries. Data governance also needs to cover security and access rights, protecting confidential data from unauthorized access and therefore ensuring compliance.
Data quality tools and metadata
As well as rules that help ensure the quality of data, organizations need to automate data processing to remove manual steps that cause inefficiencies and potential errors. Data quality tools that check and fix data, particularly for common issues (such as formatting mistakes) or processes (such as anonymizing personally identifiable information) need to be an integral part of all data processing flows. Many solutions, such as Opendatasoft, have built-in processors that can be used to improve data quality, maximizing efficiency and accuracy.
Accurate metadata is also central to data quality. Metadata is “data about data”, providing context to what a dataset contains and explaining its provenance. This helps people better understand the dataset and increases interoperability. Metadata improves data quality by providing consistent answers to questions users might have about a dataset.
Training and data culture
While data governance and tools put in place the right processes and frameworks to deliver data quality, ultimately it is vital to build a strong, confident data culture across the organization. This ensures that data is treated as a key business asset by everyone, from data creators and stewards to users. They need to be educated on its benefits, how to harness it, and the importance of using data while safeguarding privacy and compliance.
Data marketplaces and data quality
Data marketplaces enable organizations to share data at scale, creating a central, secure portal that collects and makes available all relevant data, to all users. An intuitive, self-service, e-commerce style experience makes it easy for everyone to discover and reuse data, while ensuring compliance through robust access rights management. Data marketplaces play a key role in ensuring data quality, with in-built processors to check and fix quality issues and drive data governance, strong metadata to describe datasets, and the ability for users to connect directly to data producers with feedback and queries. All of this makes data more available and builds confidence and trust in it, helping spread its use across the organization and beyond.
Want to learn how Opendatasoft can help meet your data quality challenges? Contact us to find out more and book a personalized demo.
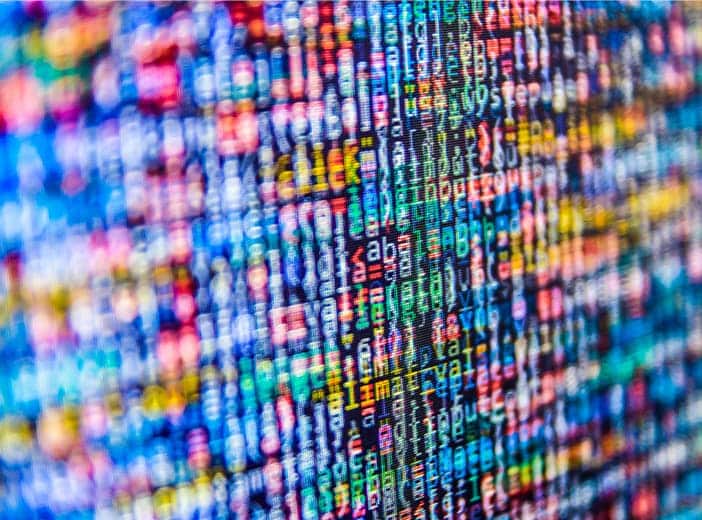
Understanding the importance of metadata and putting the right strategy in place is vital to effective data sharing and reuse via data portals to progress towards data democratization. Our comprehensive blog explains what metadata is, outlines its benefits and shares best practice for your strategy.
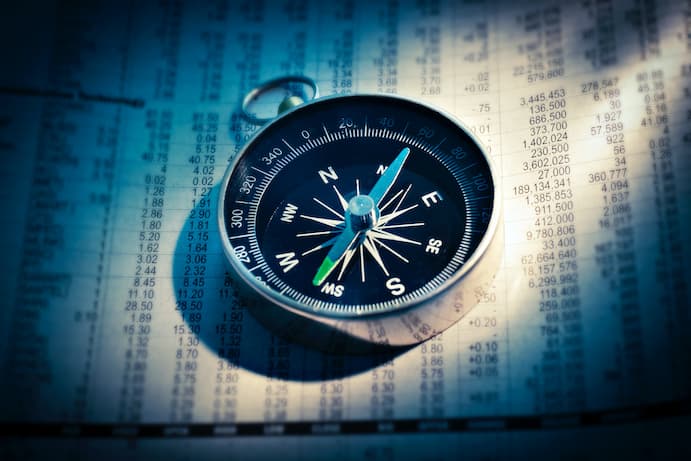
Strong data governance is vital to extend the use and value of your data across your organization and ecosystem, but also to protect it and meet regulatory obligations. We explain the benefits and challenges of data governance and share best practice advice for successfully introducing programs that will help you become a data-driven organization.
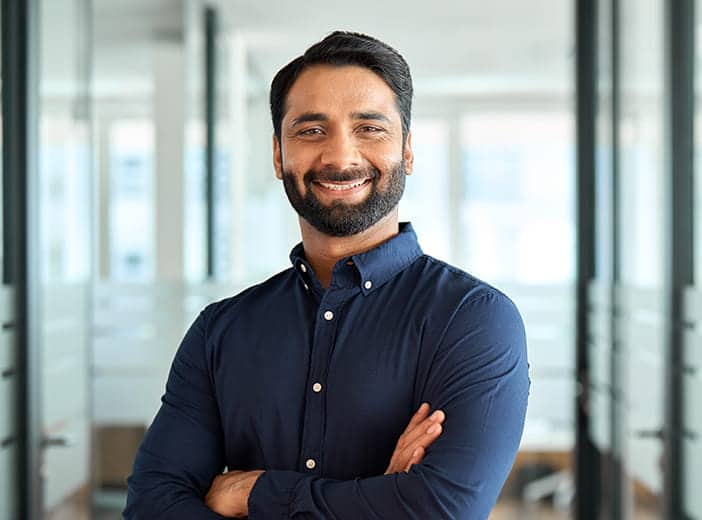
Chief Data Officers are central to organizations becoming data-centric, maximizing data sharing to ensure that everyone has immediate access to the information they need. We explore the challenges they face - and how they can be overcome with the right strategy and technology.