Glossary
Big data
Big data covers the technologies, methods and processes used to collect and analyze the enormous volumes of data now produced by organizations.
The explosion in the amount of data now available to organizations – and the value that can be gained from it – makes managing this big data a business necessity. This has accelerated the development of new data storage and analysis technologies, including artificial intelligence (AI) and machine learning (ML). We explain what organizations need to manage the transition to the big data era.
What is big data?
Big data covers the technologies, methods and processes used to collect and analyze the enormous volumes of data now produced by organizations. Harnessing big data enables better decision-making, greater innovation and increased efficiency and innovation.
Gartner defines big data in terms of the 3Vs:
- Volume: big data refers to huge volumes of data. Some organizations are now creating and storing petabytes of data. To give an idea of size one petabyte is equal to 1,000 terabytes and is the equivalent of 500 billion pages of printed text.
- Velocity: These large volumes of data are transmitted at great speed. As a result, organizations have access to data in real or near-real time. This creates difficulties in being able to process data quickly enough..
- Variety: Big data is composed of structured, unstructured or semi-structured data from a wide range of sources. It is therefore necessary to perform upstream processing to standardize these data sets.
Two other Vs have now been added to the original three:
- Value: the value that big data brings to the organization
- Veracity: data must be reliable and of high quality.
Thanks to these characteristics, big data allows organizations to solve both current and new problems.
What technology should be adopted to deal with big data?
Big data allows organizations to store a large amount of data from different sources, such as enterprise data, data from Internet of Things (IoT) sensors, geolocation data, and data from open data sources.
In order for this data to be easily accessible for all employees and to be seamlessly integrated with business applications to create new uses, organizations must have the right technology in place::
- to store data (such as data lakehouse or cloud storage solutions)
- to analyze it (through business intelligence, data science and AI)
- to share data, internally or externally (via data experience platforms and data visualizations)
What opportunities does big data offer?
Collecting and analyzing large volumes of data allows organizations in all sectors to create new opportunities. For example, they can:
- Create new services: data enables the creation of new services, which can drive new revenues or increase ecosystem collaboration. For example, Schneider Electric has built Exchange, a marketplace of data services for collaboration and networking between players in the energy sector.
- Improving productivity: companies can use big data to increase productivity and improve efficiency. For example, shipping companies are now equipping freight containers with intelligent sensors to automatically track and provide information on their contents, simplifying and speeding up supply chain processes.
- Improving the customer experience: thanks to big data, companies can gather valuable information about their target customers, and thus better understand their needs. In doing so, they are able to provide a personalized customer experience.
What are the challenges of big data?
While big data offers organizations new opportunities, it also presents a multitude of challenges in terms of turning data into real value. Without in-house expertise, the right tools and reliable, high-quality data, creating value can be hard. Organizations therefore need to focus on improving end-to-end data management, so that they can benefit from big data. They need to cover these areas:.
- Data protection: big data often contains confidential information, such as personal customer data, financial or medical information. Companies therefore have an obligation to ensure this data is kept secure and usage complies with laws such as the GDPR and CCPA.
- Data quality and interoperability: as there are many different types of data, it is imperative to define rules to ensure data quality and interoperability. Without guarantees that data is reliable and trustworthy, it cannot be used with confidence, preventing organizations from benefiting from big data.
- Data reuse: The growth of big data has led organizations to invest heavily in solutions to store and analyze data. However, often data is still trapped in silos or only available to experts. Organizations therefore need to invest in tools to make their data available to those who need it, with the ability to share it securely with the outside world.
En savoir plus
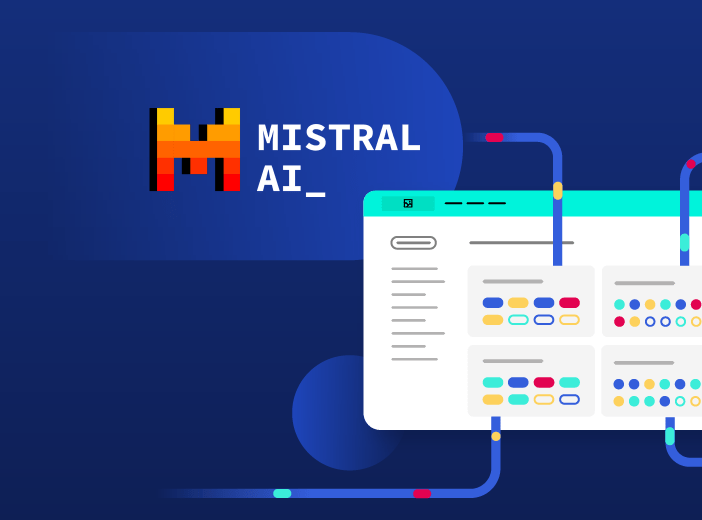
Opendatasoft integrates Mistral AI’s LLM models to provide a multi-model AI approach tailored to client needs
To give customers choice when it comes to AI, the Opendatasoft data portal solution now includes Mistral AI's generative AI, alongside its existing deployment of OpenAI's model. As we explain in this blog, this multi-model approach delivers significant advantages for clients, their users, our R&D teams and future innovation.