Glossary
Data Analyst
A data analyst collects, processes and analyzes an organization's data, ensuring data is used as a decision-making tool.
Given the rising importance of data across all sectors, organizations are increasingly employing data analysts within their teams. So, what is their role? What kind of skills do they need? How will the role change going forward?
What is the role of the data analyst?
The role of a data analyst is to collect, process and analyze an organization’s data. A data analyst uses data as a tool to support better, more informed decision-making.
While data analysts are employed across all sectors, not all organizations have the same goals. The role of the data analyst therefore varies between companies. For example, data analysts can help marketing teams understand market trends or assist sales teams in improving their performance. But they can also use data to solve environmental, social, IT and other issues.
Regardless of the goal, a data analyst generally needs to perform the following tasks:
- Creating dashboards: this provides decision-makers with an at a glance overview of its current activities and its market.
- Reporting: since data plays an important role in decision-making, data analysts create regular reports to help senior managers take more informed decisions.
- Designing and maintaining reliable and secure databases: the aim is to ensure optimal data quality from across all sources.
- Improving data processes: in order to deal with ever-increasing volumes of data, data analysts need to focus on improving process as part of an organization’s data governance strategy.
Data analysts do not work alone when performing these various tasks. They normally collaborate with IT teams, management and/or other data scientists.
What skills does a data analyst need to have?
Along with a perfect command of data, data analysts must first of all have a passion for mathematics, business intelligence and big data.
On the technical side they must be proficient in the following types of IT tools:
- Programming languages for collecting, cleaning, analyzing and visualizing data.
- Databases: such as NoSQL and relational databases.
- Data mining, cleaning and manipulation: extracting, enhancing and masking information to enable it to be ready for analysis.
- Excel: even though there are now many analytics tools that provide automated data processing, data analysts must have advanced modeling and analysis skills in Excel.
- Machine learning: this is a real plus, but is not a necessary prerequisite for becoming a data analyst.
In addition to these technical skills, data analysts must also have excellent communication skills, attention to detail, business understanding, creative thinking and analytical vision.
Given that the range of skills required to be a data analyst is wide, few people possess all of them. That means that data analysts are very much in-demand, commanding large salaries. This holds back data democratization, as they are the gatekeepers of data, potentially acting as a bottleneck between users and data..
What is the difference between a data analyst and a data scientist?
All data scientists and data analysts are data experts. The major difference is that the primary role of a data analyst is to analyze already-existing data. By contrast, a data scientist may be asked to design new data sources and identify important value-added insights.
How is the role of the data analyst evolving?
As technologies continue to develop, the tasks performed by data analysts are constantly evolving. They must analyze ever larger, more scattered and more varied volumes of data, using new techniques such as artificial intelligence and machine learning.
The data analyst’s goal is to set up advanced mathematical predictive models to analyze data, and to extract and present this data in a way which is readable in order to make decision-making easier at all levels of the organization.
This task can quickly become time-consuming, which is why it is important for companies to widen access to data. They need to implement easy to use data experience solutions that enable non-expert employees to work with data on a day-to-day basis, thus allowing data analysts to concentrate on high value-added tasks.
Data analysts therefore help organizations make the best strategic decisions in order to increase their competitiveness.
Learn more
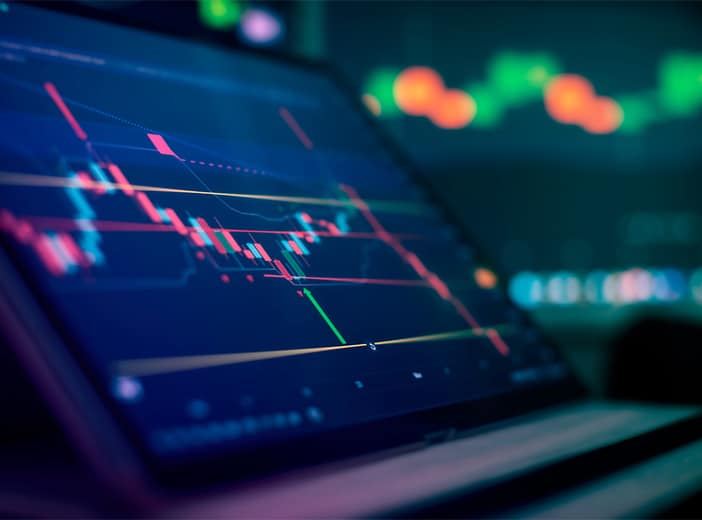
Generating value from data through a data product marketplace
Data product marketplaces empower businesses and data leaders with the ability to industrialize data sharing and consumption to create data value. We look at the key foundations you need to put in place to ensure your data product marketplace successfully generates value, increases usage and drives ROI.
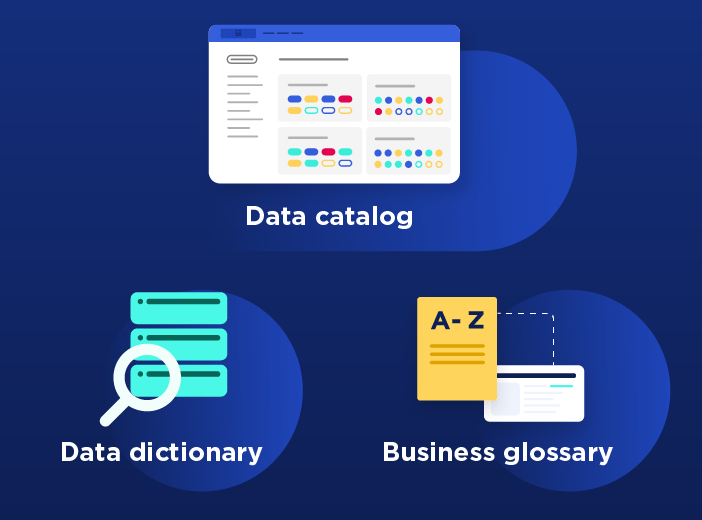
What are the differences between a business glossary, a data dictionary and a data catalog?
Organizations face an unprecedented explosion in data volumes. However, this information is scattered across the business, in multiple formats, making it difficult to organize, analyze and share. How can organizations gain control over their data and use it effectively?
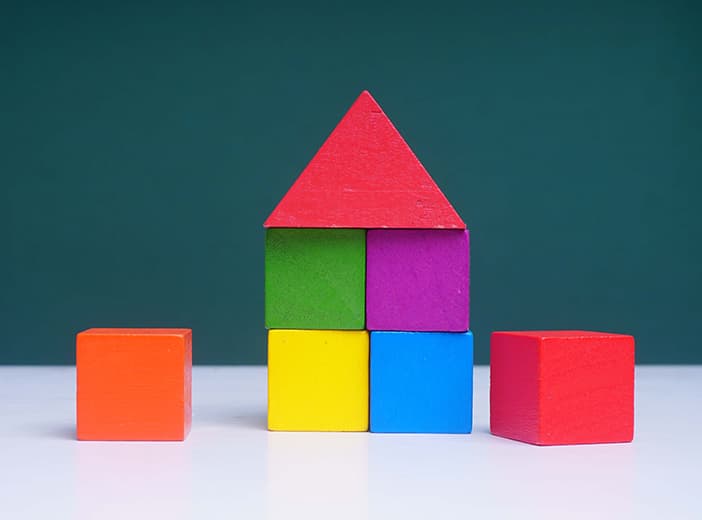
What is a data product marketplace?
To meet their need to increase data consumption and maximize value, businesses are increasingly implementing centralized, self-service data product marketplaces. We look at what they are, and how they deliver value for employees, the business, data teams and data leaders.