Glossary
Data cleansing
Data cleansing (or data cleaning) is the process of identifying and fixing incorrect, incomplete, duplicate, unneeded, or invalid data in a data set.
What is data cleansing?
Data cleansing (also called data cleaning or data scrubbing) involves identifying and fixing incorrect, incomplete, duplicate, unneeded, or otherwise erroneous data in a data set. This normally takes place during the data preparation phase, and ensures that data is accurate and actionable when shared, analyzed, and reused.
It is separate to data transformation, when data is enriched with additional information and datasets, such as by adding geographical information. Data cleansing normally occurs before data transformation in the data preparation phase.
Data cleansing can be carried out manually, updating and correcting records, or by using data cleansing tools that automate all or part of the process.
The terms data cleansing, data cleaning and data scrubbing are normally used interchangeably. However, in some cases, data scrubbing refers solely to removing (rather than identifying) duplicate, bad, unneeded or old data from data sets.
Why is data cleansing important?
It is fundamental to ensuring high data quality, so that information is accurate, consistent and able to be used with confidence across the organization and beyond. Without effective data cleansing, business decisions may rely on inaccurate data, preventing organizations becoming data-driven. As the saying goes when it comes to data “garbage in, garbage out.”
What are the benefits of data cleansing?
With data central to business operations and to transparency across wider society, ensuring that data is accurate and actionable is vital. Data cleansing therefore provides 5 specific benefits:
- Better decision-making. Being able to make faster, better informed decisions is essential for business success. If data is not cleansed errors that impact the accuracy of decision-making can occur. This is a particular issue when data is used with AI algorithms, without human oversight.
- Greater confidence and trust. High quality data is at the heart of data democratization. Employees and citizens need to trust that the data they are accessing is accurate, otherwise they simply will not use it.
- Time savings. Cleansing data at the preparation stage guarantees accuracy when it is shared and used. This saves time and resources, as it is fixed once, at source.
- Greater productivity. Higher quality data means that employees are able to focus on decision-making, rather than looking for mistakes in their datasets, increasing their productivity.
- Reduced data costs. Cleansing data removes duplicates and inaccurate records, shrinking storage requirements and leading to faster processing times for datasets.
What are the characteristics of clean data?
Data quality can be measured using these characteristics:
- Accuracy. Is the data correctly representing what it is measuring?
- Consistency. Is data consistent across different datasets? For example, is a customer address the same between CRM and billing systems?
- Validity. Does the data meet set parameters or rules? For example, is a telephone number in the correct format?
- Completeness. Are there gaps in the data and can this be fixed with data from other sources?
- Uniformity. Has the data been collected and represented using the same units and scales? For example, are measurements in inches and feet or meters and centimeters?
Data teams will use data quality metrics to measure these characteristics within datasets, as well as calculating overall error rates in datasets.
What types of errors does data cleansing fix?
Examples of common errors that can be discovered and fixed within the data cleansing process include:
- Missing or invalid data – spotting gaps in fields or data that is in the wrong format (such as a numerical value in a text field).
- Typos – misspellings or other typographical errors
- Inconsistencies – common fields (such as addresses or names) that are formatted or described differently between datasets.
- Duplicates – multiple records relating to the same thing (such as a customer). This often occurs when different datasets are merged.
- Irrelevant data – data that is not needed by the organization. For example, a municipality may import a state-wide dataset, but only want to use data related to itself.
How does the data cleansing process work?
While the process will vary depending on the organization, the tools used and the data itself, it normally covers these 5 steps:
1. Data auditing to inspect data and identify anomalies and issues, which are then dealt with in the order below
2. The removal of duplicate or irrelevant data/records
3. Fixing structural errors, such as inconsistencies between fields
4. Handling any missing pieces of data, such as by comparing with other data sources
5. Verification to check that all errors have been removed and that the data meets internal data quality standards
Depending on the size and complexity of datasets, the data cleansing process will use a combination of automated tools and manual, human oversight and input.
Learn more
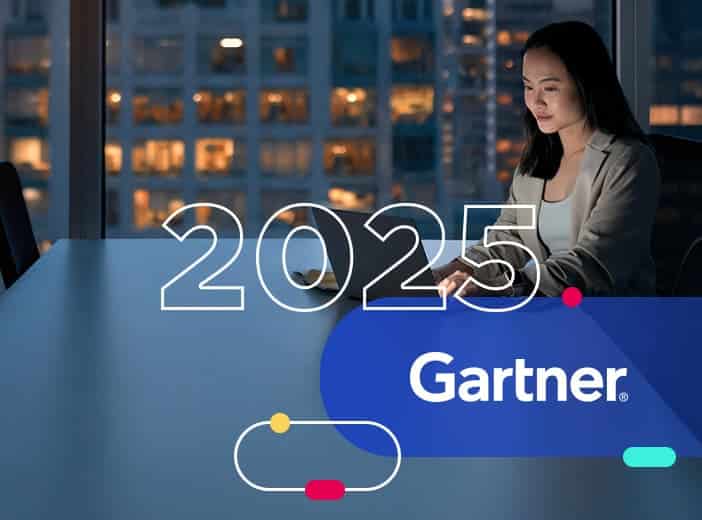
How data leaders deliver value – Gartner’s 2025 Leadership Vision
The role of the data leader has never been more vital, but they face growing pressure to increase their business value and support AI initiatives. Based on a new Gartner guide, we outline the vision to follow to maximize success for their organization.
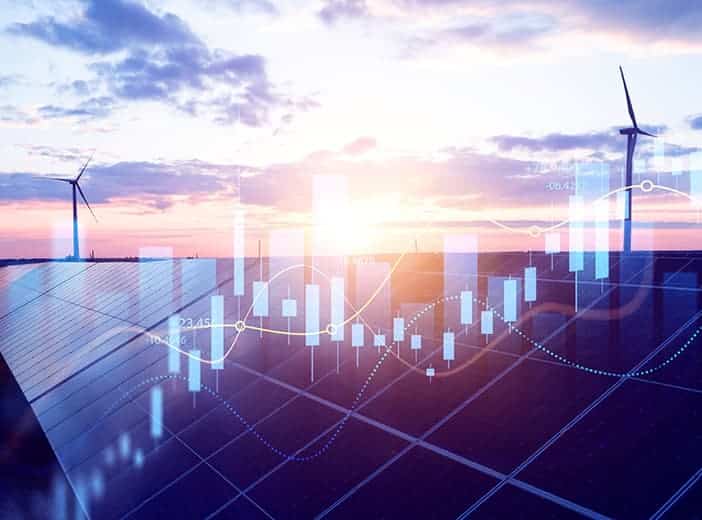
Driving forward data sharing in the energy sector
How can energy and utility companies successfully share their increasing volumes of data with all stakeholders to help deliver on net zero goals? Our Energy User Club brought together leading utilities to discuss best practice around scaling data sharing and value.
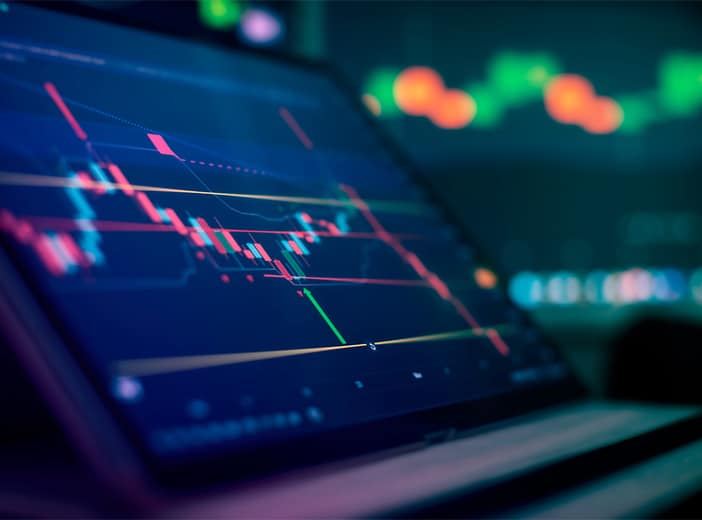
Generating value from data through a data product marketplace
Data product marketplaces empower businesses and data leaders with the ability to industrialize data sharing and consumption to create data value. We look at the key foundations you need to put in place to ensure your data product marketplace successfully generates value, increases usage and drives ROI.