Glossary
Data sourcing
Data sourcing is the systematic, methodological process of collecting raw data from inside and outside an organization.
What is data sourcing?
Data sourcing is the systematic, methodological process of collecting raw data, from multiple sources across an organization and beyond. It is the first step in any data management process, and using this data enables organizations to understand performance, make better-informed decisions, test hypotheses and share data internally and externally.
Data sourcing covers multiple types of information, both quantitative and qualitative, including:
- Data created by systems in the course of their operations (such as IoT sensors, machinery, connected cars)
- Data captured through human behavior, such as while a customer is navigating a website
- Data entered into systems by customers/consumers, such as filling in online forms
- Data entered into systems by staff, such as while on the phone to customers in a contact center
- Data generated by business systems, such as sales, CRM, or accounting solutions
- Data captured by researchers directly from survey interviews or focus groups
- Data gathered from third parties, such as partners or data brokers
What is the data sourcing process?
We live in a world where data is being created at an exponentially increasing rate. Therefore, it is important to take a systematic approach to data sourcing, rather than simply collecting data without a plan or methodology. Following this process is recommended:
- Identify what you are aiming to achieve. This will affect which data you collect and where you collect it from
- Select the right datasets to collect. Understand which data sources you are going to gather to achieve your goals and find out who owns the data
- Quality check the data before beginning collection. Work with the data owner to ensure that the data meets your needs and has the level of granularity required
- Create a documented process for data sourcing. This should include how often data will be collected, where it will be routed to, how it will then be used, and the security that will be in place to protect it
- Collect data. Automate the process of collecting data, but ensure that there is rigorous checking for errors to ensure it is in the right format and of high quality
- Regularly review the entire process, particularly if there are any changes in the data source itself
What are the challenges to effective data sourcing?
As it is the first step in the data management process, it is essential that data sourcing is carried out correctly and systematically.
Organizations therefore need to overcome these key challenges to ensure their data sourcing delivers value:
- Ensure data quality. Data must be accurate and reliable, otherwise, results and decisions taken later in the process will be unreliable. When sourcing data ensure that:
- It is standardized and is in the correct format, with descriptions of each field so that it can shared and combined later in the process
- All relevant data is captured, without gaps or errors
- You understand key parameters around the data, such as the frequency of its creation and its granularity
- It is consistent, using the same units (such as for time, speed, or weight)
- Ensure data is fit for purpose. Data may be generated for one purpose (such as running an industrial machine), but then used for other reasons (such as calculating the overall efficiency of a factory). Ensure that the data you gather is a good fit for your needs.
- Ensure data is secure. Reduce compliance risk by ensuring data sourcing is safe, meets regulations such as the GDPR/CCPA, and protects personally identifiable information (PII)
Learn more
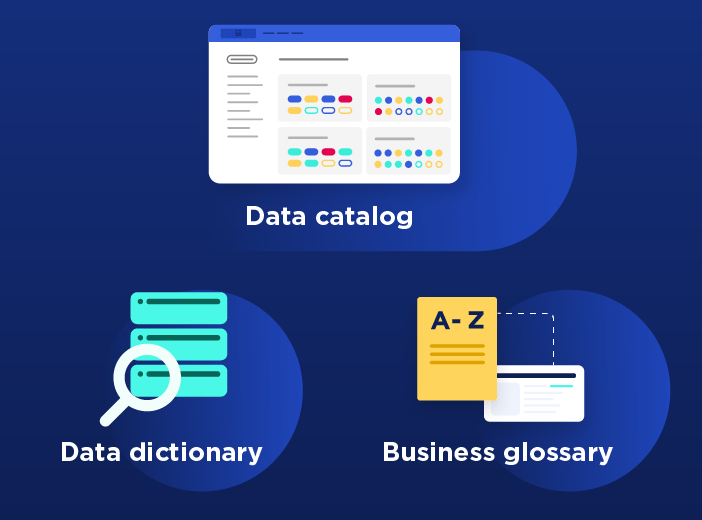
What are the differences between a business glossary, a data dictionary and a data catalog?
Organizations face an unprecedented explosion in data volumes. However, this information is scattered across the business, in multiple formats, making it difficult to organize, analyze and share. How can organizations gain control over their data and use it effectively?
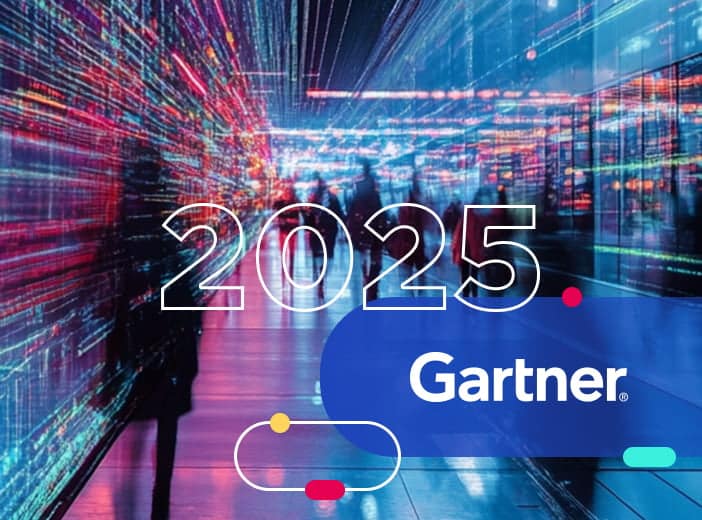
2025 data leader trends and the importance of self-service data – insights from Gartner
Growing data volumes, increasing complexity and pressure on budgets - just some of the trends that CDOs need to understand and act on. Based on Gartner research, we analyze CDO challenges and trends and explain how they can deliver greater business value from their initiatives.