Data Virtualization
What is data virtualization?
Data virtualization brings together data from multiple, disparate sources across the organization, in real-time, in a single, virtual location. Data is not physically moved from its source location but instead displayed through data virtualization middleware, which acts as a virtual data layer (or semantic layer). This means that users are able to consume data without needing to be aware of its type or storage location.
Data virtualization therefore enables faster, cheaper access to up-to-date data, particularly for applications such as analytics. Thanks to integrated governance and security features, data virtualization ensures that the data shared with business users is consistent, high quality, and protected.
How does data virtualization work?
Data virtualization follows a three step process:
- Connect: connecting to any data source (either on-premise or cloud), such as databases, applications, cloud storage or data warehouses
- Combine: combining all types of data, including structured and unstructured
- Consume: enabling business users to consume data through reports, dashboards, portals, and applications.
What is data virtualization used for?
Data virtualization is primarily used for:
- Business intelligence and analytics – bringing data together from across the business in real-time to enable querying and reporting, no matter how complex the underlying data architecture is.
- Self-service data access – enabling business users to quickly access virtualized data in order to run reports and measure performance.
- Application development – reducing the coding required to create new applications by simplifying the process of connecting to data sources.
- Real-time data backups – enabling faster data/system recovery.
What is the difference between data virtualization and data integration?
Both data virtualization and data integration combine disparate sources of data and make them available to users through a single perspective.
The major difference is that data integration does this by physically taking all data, changing its format and then loading it into a single location, while data virtualization achieves this virtually, without moving the underlying data.
What are the benefits and drawbacks of data virtualization?
The benefits of data virtualization
- Speed – as data is accessed wherever it is stored, access is much faster and simpler, with data potentially available in real-time.
- Efficiency – as data is not moved to separate systems, it reduces costs in terms of hardware, software, governance, and time. It is much cheaper than creating and populating a separate repository for all of an organization’s data.
- Security and governance – data virtualization provides a centralized approach to data security and governance across the organization. It reduces the risk of errors as data remains within its original source system.
- Self-service access – data can be accessed by any data consumer, without requiring technical skills.
- Scalability – new data sources can be quickly added without the need for time-consuming ETL processes.
- Quality – data virtualization eliminates redundant or duplicate data, increasing reliability and efficiency.
What are the disadvantages of data virtualization?
- Limited to simple data processing – while data virtualization brings data together, it does this through simple processing rules. It cannot handle complex data transformations, which require data integration/ETL.
- No support for batch data movement – data remains virtualized and is not moved/transformed to new systems, such as data warehouses.
- Poor performance for operational data – data virtualization works well for analytics queries. However, it performs less well around moving or virtualizing large volumes of operational data where latency can be a major issue.
- No historical analysis of data – as queries are carried out on the fly, there is no record of historical queries for comparative or repeat analysis.
- Relies on source systems – unlike in a data warehouse, where data is physically moved, data virtualization relies on source systems to be online/operational to be able to access their data.
- Single point of failure – if the virtualization server has an issue, it prevents any data being made available to other systems, increasing risk by acting as a single point of failure.
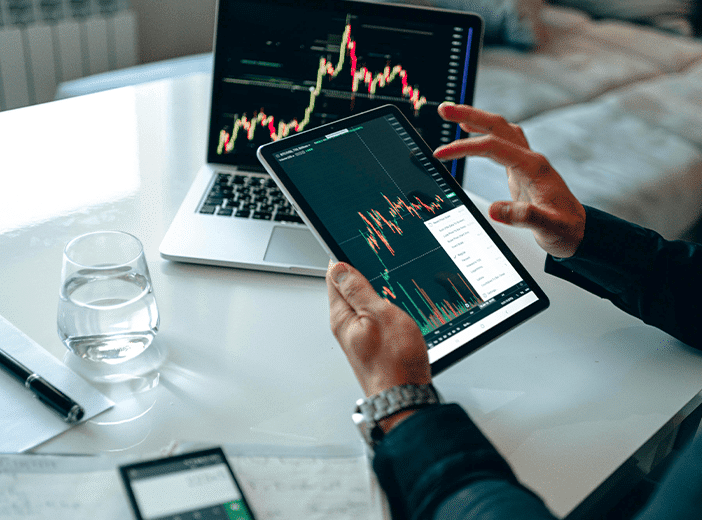
What is agentic AI and how does it help increase data consumption? Our Q&A blog explains the current state of AI, and how Opendatasoft is innovating to drive forward its impact for customers.
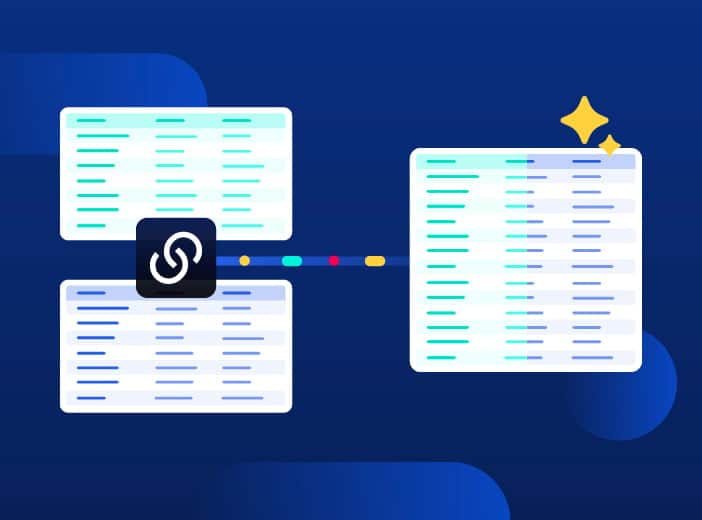
Enriching your data is a key step in creating relevant insights and analysis that drives value. However, when it comes to using massive reference sources such as national company databases, detailed weather data or geographic/administrative boundary datato enrich your data, technical limitations often become a challenge.
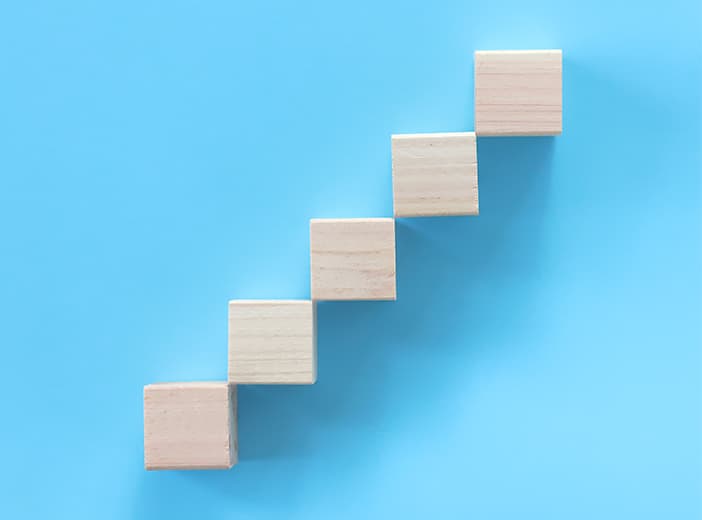
Data products are central to increasing data consumption across the organization. But how can you ensure your data product program delivers lasting value? We explore the latest best practice from McKinsey, designed to scale data product creation and usage.